Get a Free WorkTrek Demo
Let's show you how WorkTrek can help you optimize your maintenance operation.
Try for freeWhat is the Mean Time Between Failure (MTBF), and how does it relate to equipment reliability? It tells us how long a machine or system typically runs before it breaks down. MTBF is the average time between failures of a repairable system during normal operation.
Engineers and maintenance teams use MTBF to plan repairs and predict when parts might fail. A higher MTBF means a system is more reliable and breaks down less often, helping companies save money on repairs and avoid unexpected downtime.
Source: WorkTrek
MTBF is useful for many types of equipment, from factory machines to computer servers. It helps businesses make smarter choices about when to replace parts or upgrade systems.
By tracking MTBF, companies can improve their maintenance strategies and keep their operations running smoothly.
What is MTBF
Mean Time Between Failures (MTBF) is a key metric in reliability engineering. It helps predict equipment performance and plan maintenance schedules. MTBF impacts product design, quality control, and operational efficiency.
Definition and Fundamentals
MTBF stands for Mean Time Between Failures. It measures the average time a repairable system operates between failures. The metric is calculated by dividing the total operating time by the number of failures.
For example, if a machine runs for 1000 hours and fails twice, its MTBF is 500 hours. A higher MTBF indicates better reliability.
Engineers use this data to improve designs and maintenance plans.
MTBF applies to repairable systems. Mean Time To Failure (MTTF) is used instead for non-repairable items.
How to Calculate MTBF
The MTBF formula is simple but powerful. It’s calculated by dividing the total operational time by the number of failures:
MTBF = Total Operational Time / Number of Failures
Source: WorkTrek
For example, if a machine runs for 1000 hours and fails five times, its MTBF is 200 hours.
This formula assumes the system is repairable and can be returned to service after each failure. Mean Time To Failure (MTTF) is used for non-repairable items.
It’s important to note that MTBF is an average. Some failures may occur sooner, while others may happen much later than the calculated MTBF.
Common Pitfalls in MTBF Calculation
Several mistakes can lead to inaccurate MTBF calculations:
- Ignoring partial failures or minor issues
- Including planned downtime in operational hours
- Not considering the system’s age
- Using too small a sample size
Source: WorkTrek
Another common error is applying MTBF to non-repairable items. For these, MTTF should be used instead.
Some organizations focus solely on MTBF without considering other reliability metrics. A holistic approach that includes metrics like Mean Time To Repair (MTTR) provides a more complete picture of system reliability.
Data Collection and Analysis
Accurate MTBF calculation relies on thorough data collection. Organizations need to track:
- Total operational hours
- Number of failures
- Dates and times of failures
- Repair times
Illustration: WorkTrek / Data: Deloitte
Maintenance management systems often automatically collect this data. Regular equipment inspections and operator reports also provide valuable information.
Analysis should consider the operating conditions and environment. Factors like temperature, humidity, and usage intensity can affect failure rates.
It’s crucial to define failure clearly, and this definition should be consistent across all data collection efforts.
Differences Between MTBF, MTTF, and MTTR
MTBF, MTTF, and MTTR are related but distinct concepts:
- MTBF: Applies to repairable systems. Measures average time between failures during normal operation.
- MTTF (Mean Time To Failure): Used for non-repairable items. Represents the average lifespan before failure.
- MTTR (Mean Time To Repair): Measures the average time needed to fix a failed system.
Source: WorkTrek
These metrics work together to give a complete picture of system reliability. For example, a product with high MTBF and low MTTR would be available.
Engineers use these measures to optimize maintenance strategies and improve overall system performance.
Importance of MTBF in Reliability Engineering
MTBF plays a crucial role in assessing equipment reliability. It helps engineers:
- Predict failure rates
- Plan preventive maintenance
- Compare different designs or products
- Set reliability targets
- Estimate spare parts needs
Source: WorkTrek
A high MTBF in manufacturing can lead to less downtime and lower costs. For consumer products, it can mean fewer repairs and higher customer satisfaction.
MTBF data guides warranties, service contracts, and product lifecycle management decisions. It’s essential for industries where failures, like aerospace or healthcare, can be costly or dangerous.
MTBF in Product Design and Development
MTBF plays a key role in creating reliable products. It guides design choices, shapes maintenance plans, and helps meet reliability goals.
Incorporating MTBF into Design
Designers use MTBF to make products that last longer. They pick parts with high MTBF values to boost overall product life.
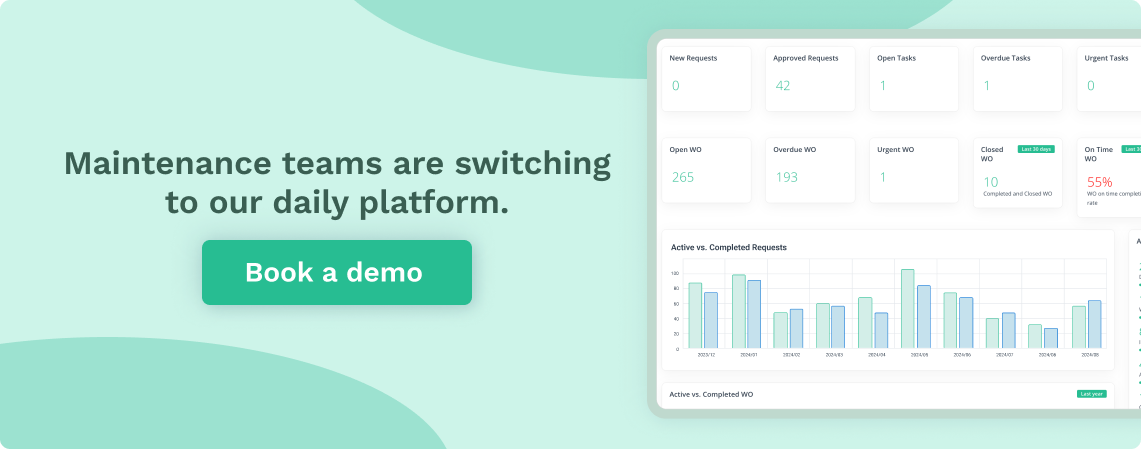
Reliability calculations help find weak spots in designs. Teams can then fix these issues early on.
MTBF targets guide choices about materials and parts. Designers may use stronger materials or add backup systems to achieve MTBF goals.
Testing is key to checking if products meet MTBF targets. Teams run stress tests and long-term trials to verify reliability claims.
MTBF and Preventive Maintenance
MTBF helps plan when to do maintenance. It shows how often parts might fail.
Teams use MTBF to set maintenance schedules. They replace parts before they’re likely to break.
Source: WorkTrek
This cuts down on sudden breakdowns. It also makes products last longer.
MTBF data helps decide which parts to keep in stock. It shows which items might need replacing soon.
Smart maintenance based on MTBF can save money. It reduces downtime and extends product life.
Role of MTBF in Design for Reliability (DfR)
Design for Reliability (DfR) uses MTBF to make products that last. It’s about building reliability into products from the start.
DfR teams set MTBF goals early in design. They then work to meet or beat these targets.
They use tools like Failure Modes and Effects Analysis (FMEA) to find potential issues, which helps prevent problems before they start.
MTBF guides choices in DfR. It might lead to using more durable parts or adding safety features.
DfR also uses MTBF to compare design options. The choice that offers the best MTBF often wins.
MTBF and Risk Management
Mean Time Between Failure (MTBF) plays a key role in risk management for industrial and electronic systems. It helps predict equipment reliability and informs maintenance planning to reduce downtime risks.
MTBF as a Risk Indicator
MTBF serves as an important measure of system reliability. A higher MTBF suggests lower failure risk, while a lower MTBF indicates higher risk.
Companies use MTBF data to:
- Identify high-risk components
- Plan preventive maintenance schedules
- Estimate spare parts inventory needs
- Calculate potential downtime costs
Source: Infosec-Reading
By tracking MTBF trends over time, organizations can spot declining equipment performance early and take action before failures occur.
MTBF also helps compare reliability between different equipment options. When choosing new systems, a higher MTBF often means lower long-term risk.
Integrating MTBF with Risk Assessment
MTBF data enhances broader risk assessment efforts. It provides concrete numbers to support risk analysis and decision-making.
Risk managers can use MTBF to:
- Quantify the likelihood of equipment failures
- Estimate the financial impact of potential downtime
- Prioritize risk mitigation efforts
MTBF calculations factor into Life Cycle Cost (LCC) analysis. This helps predict long-term operational risks and costs.
Combining MTBF with metrics like Mean Time To Repair (MTTR) gives a fuller picture of risk. Together, they show both failure frequency and recovery time.
Regular MTBF reviews allow companies to adjust their risk management strategies. As equipment ages or conditions change, MTBF helps keep risk assessments up-to-date.
Case Studies
Mean Time Between Failures (MTBF) is a key metric used across various sectors to measure system reliability. Its application and significance vary depending on the specific industry and the critical nature of the equipment involved.
MTBF for Data Centers
Regarding large server farms and data centers, MTBF plays a huge role in understanding when to maintain or replace equipment. Heat, usage, and even human error can cause equipment failure.
Illustration: WorkTrek / Data: The Raw Review
A great example of a company that employs this practice while publicly publishing its data is BackBlaze. It has tracked failure rates across various hard drives for several years and published the results on its website.
This data has been invaluable for the company and, due to their generosity, to the rest of the data center industry.
MTBF in Aerospace and Defense
MTBF is critical for safety and mission success in aerospace and defense. Aircraft manufacturers use MTBF to design reliable systems and plan maintenance schedules.
General Electric Transportation Systems is using data analysis to improve its products. As highlighted in this detailed case study by NASA, it continuously collects customer field reliability data and stores it for analysis.
They use this data to continuously adjust MTBF calculations for their equipment in the field, which helps greatly reduce equipment failure.
Challenges in Applying MTBF
MTBF analysis has hurdles in real-world applications. Issues arise from the metric’s inherent limitations and how people interpret the data.
Limitations of MTBF Analysis
MTBF calculations assume constant failure rates, which rarely occur in practice. This can lead to inaccurate predictions for complex systems.
Maintenance managers may struggle to account for varying operating conditions that affect failure rates. Environmental factors, usage patterns, and maintenance practices can all impact system reliability.
MTBF also doesn’t consider the severity of failures. A minor glitch and a catastrophic breakdown are treated equally in the calculation.
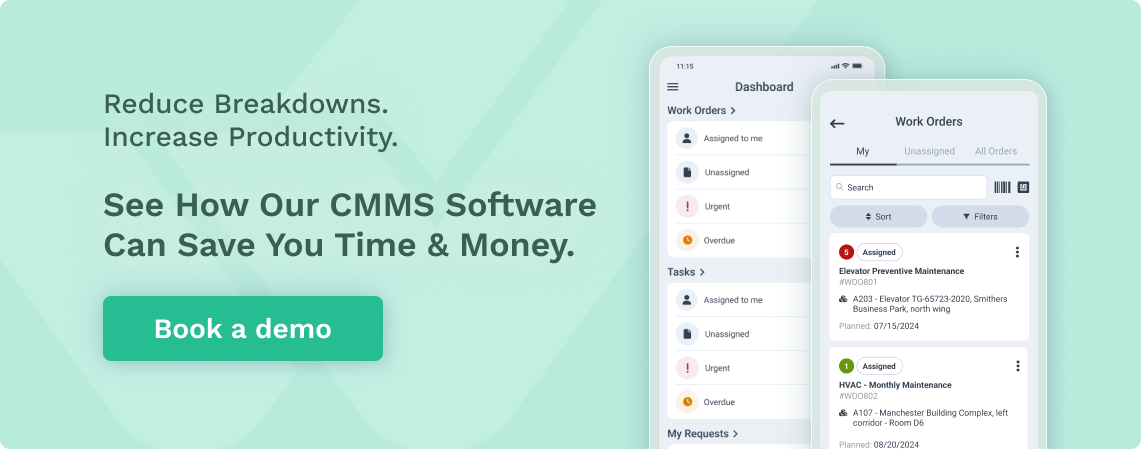
MTBF can be misleading for repairable systems. It doesn’t distinguish between the time to first failure and subsequent failures after repairs.
Misinterpretation of MTBF Data
People often misunderstand MTBF as a guarantee of failure-free operation. It’s an average that doesn’t predict specific failure times.
Some mistakenly believe MTBF represents a component’s useful life, which can lead to premature replacements or delayed maintenance.
Reliability engineers may face challenges explaining MTBF to non-technical stakeholders. The concept of an average time between failures can be counterintuitive.
Comparing MTBF values between different types of systems or components can be problematic. Without context, these comparisons may lead to flawed decision-making.
Improving MTBF
Boosting Mean Time Between Failures (MTBF) is key for better equipment reliability. Companies can use several methods to extend the time between breakdowns and increase overall system performance.
Strategies for Enhancing MTBF
Preventive maintenance programs are a top way to improve MTBF. These programs help catch issues before they cause failures. Regular checks and part replacements can stop many problems.
Illustration: WorkTrek / Data: FinancesOnline
Training staff is also vital. Workers who know how to use and care for equipment properly can help avoid breakdowns. This includes teaching proper startup and shutdown methods.
Another important strategy is using high-quality parts. Better parts often last longer and work more reliably. While they may cost more upfront, they can save time by reducing failures.
Data analysis can reveal patterns in equipment failures. By studying this info, companies can spot weak points and fix them before they cause problems.
Role of Quality Control
Strong quality control helps boost MTBF by ensuring all parts and processes meet high standards. This starts with careful supplier selection. It is crucial to choose vendors who provide reliable parts.
Incoming inspection of parts and materials helps catch defects early, preventing the use of faulty components in equipment.
Regular testing during production can spot issues before products are finished. This allows for quick fixes, improving overall quality.
It’s key to set clear quality standards and ensure they’re followed. This applies to both the manufacturing process and the finished products.
Impact of Technological Innovations
New tech can significantly improve MTBF. Sensors and Internet of Things (IoT) devices can track equipment health in real-time. This allows for predictive maintenance, catching issues before they cause failures.
Advanced materials can make parts more durable. For example, new alloys or composites might resist wear better than traditional materials.
Improved design software lets engineers create more reliable products. They can test designs virtually, spotting potential weak points before anything is built.
Artificial intelligence and machine learning can analyze vast amounts of data. This helps predict when failures might occur, allowing for proactive maintenance.
Future Trends in MTBF Analysis
MTBF analysis is evolving with new technologies and methods. The future of MTBF will likely focus on more accurate predictions and real-time monitoring.
Machine learning and AI will play a big role. These tools can spot patterns in data that humans might miss. This could lead to better failure predictions and longer equipment life.
IoT devices will change how we gather data for MTBF calculations. Sensors can track equipment performance in real time, creating a constant data stream that will make MTBF estimates more precise.
Predictive maintenance will become more common. Instead of fixed schedules, maintenance will happen when it’s truly needed. This could reduce downtime and save money.
Illustration: WorkTrek / Data: Brickclay
Digital twins may also impact MTBF analysis. These virtual models of physical assets can simulate different scenarios. This could help predict failures before they happen in the real world.
Cloud computing will make MTBF data more accessible. Teams can share and analyze information from anywhere. This could lead to better decision-making across organizations.
The focus may shift from just measuring the time between failures. New metrics might look at the impact of failures on the whole system. This could give a more complete picture of reliability.
Conclusion
In conclusion, Mean Time Between Failures (MTBF) remains a vital metric in reliability engineering, helping organizations enhance equipment performance, optimize maintenance schedules, and reduce operational risks.
As technology advances, the future of MTBF analysis will likely see greater integration with predictive maintenance, AI, and IoT, leading to more accurate predictions and proactive strategies.