What is Smart Manufacturing?
Smart manufacturing integrates advanced digital technologies, data analytics, and interconnected systems to create an intelligent, self-monitoring, and highly adaptive production environment.
From a maintenance standpoint, it’s a revolutionary approach in which machines and systems can predict their maintenance needs. They can also communicate with each other and optimize their performance in real-time. All of this can help minimize downtime and maximize resource efficiency.
Smart manufacturing refers to implementing cutting-edge computing and technology to optimize processes.
Core Components of Smart Manufacturing
Predictive Maintenance Systems:
In smart manufacturing solutions, sensor data networks and machine learning algorithms transform traditional scheduled maintenance into predictive maintenance.
For example, at a Boeing assembly facility, vibration sensors on critical equipment continuously monitor bearing health, automatically scheduling maintenance before failures occur.
This has reduced unplanned downtime by up to 70% in some production lines and improved the overall production processes.
Digital Twin Technology:
Maintenance managers use digital twins—virtual replicas of physical assets—to simulate and optimize maintenance procedures.
Siemens employs digital twins in its gas turbine facilities to test different maintenance scenarios without risking actual equipment.
Maintenance teams can perfect their procedures virtually before executing them on physical assets.
Internet of Things (IoT) Integration
Unlike traditional manufacturing processes, smart sensors throughout the manufacturing floor create an interconnected network of equipment.
At Volkswagen’s smart factories, thousands of IoT sensors monitor everything from tool wear to environmental conditions.
Maintenance managers receive real-time alerts about potential issues and can address problems before they impact production.
Artificial Intelligence and Machine Learning
AI systems analyze vast amounts of operational data to identify patterns and predict maintenance needs.
For instance, Intel’s semiconductor fabrication plants use AI to analyze microscopic variations in equipment performance, predicting potential failures up to weeks in advance and reducing maintenance costs by 25%.
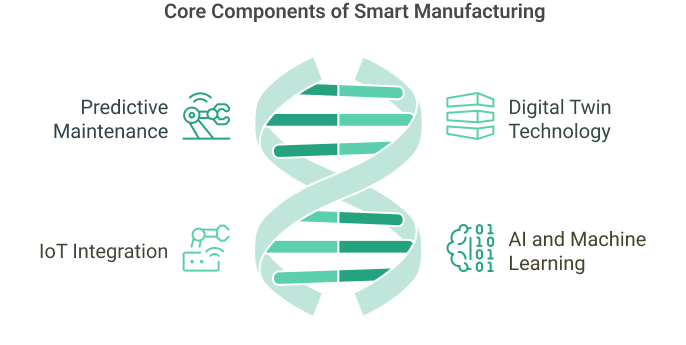
Real-World Impact on Maintenance Operations
Proactive Maintenance Planning
Instead of reactive maintenance, managers can now plan interventions based on actual equipment conditions. A paper mill in Finland implemented smart sensors on their rolling equipment, reducing emergency maintenance by 60% through early detection of alignment issues and bearing wear.
Resource Optimization
Smart manufacturing systems help optimize maintenance resource allocation. At a Schneider Electric smart factory, AI-powered scheduling ensures maintenance staff and parts are available exactly when needed, improving labor efficiency by 20%.
Knowledge Management
Advanced systems capture and preserve maintenance expertise. At Lockheed Martin, augmented reality systems guide technicians through complex maintenance procedures, reducing training time and ensuring consistent quality across maintenance operations.
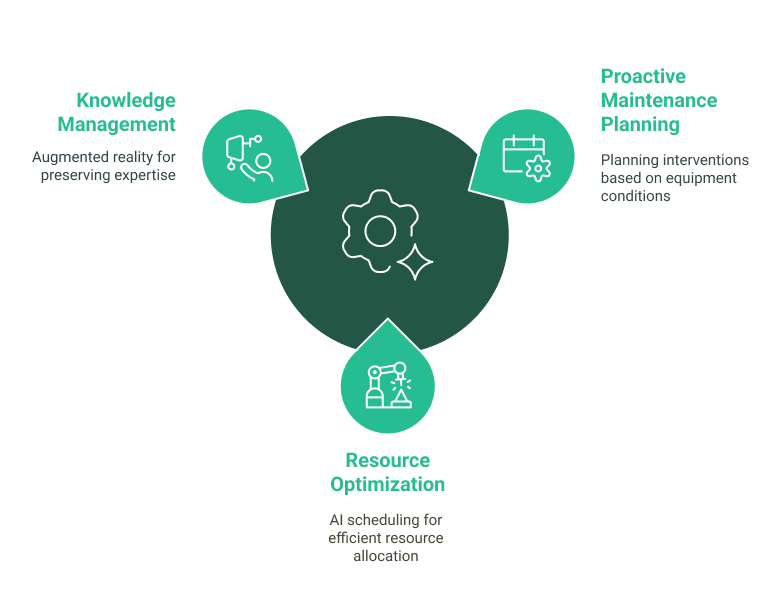
Implementation Considerations for Maintenance Managers
Infrastructure Requirements
Successful smart manufacturing practices require robust network infrastructure, real-time data, secure data storage, and reliable sensor systems. Maintenance managers must ensure their facilities can support these technological requirements.
Staff Training
Maintenance teams need new skills to work with digital tools and interpret data analytics. Companies like Toyota invest heavily in training programs to help maintenance staff transition from traditional to smart manufacturing environments.
Integration Challenges
Legacy equipment often needs retrofitting with smart sensors and controls to support smart manufacturing initiatives. One example of smart manufacturing is a pharmaceutical manufacturer gradually upgrading its equipment over three years, starting with critical systems to manage costs and minimize disruption.
Return on Investment
While initial implementation costs can be significant, smart manufacturing typically shows strong ROI through reduced downtime and maintenance costs.
For example, a General Electric aircraft engine facility reported a 60% reduction in unplanned downtime within two years of implementing smart manufacturing systems.
Future Trends for Maintenance
The evolution of smart manufacturing continues with emerging technologies like 5G networks enabling faster data transmission and more precise control.
Quantum computing and related technologies may soon allow for even more complex predictive maintenance models, while advanced robotics could automate many routine maintenance tasks.
Smart manufacturing represents a fundamental shift in how maintenance managers approach their role, moving from reactive problem-solving to proactive system optimization.
This transformation requires new skills, investments in technology, and a different mindset. Still, the efficiency, reliability, and cost savings benefits make it an essential evolution in modern manufacturing.
Historical Evolution of Smart Manufacturing
The Early Foundations (1950s-1970s):
The roots of smart manufacturing can be traced back to General Motors’ introduction of programmable logic controllers (PLCs) in 1968.
These early automated control systems marked the first step toward digitizing manufacturing processes.
Maintenance remained largely reactive during this period, with fixed schedules based on time rather than equipment conditions.
Companies like Toyota began developing their Total Productive Maintenance (TPM) system, which later became a crucial foundation for smart manufacturing principles.
The Digital Revolution (1980s-1990s)
The 1980s brought the widespread adoption of computer-integrated manufacturing (CIM). IBM and other computer manufacturers began introducing networked systems that could connect different parts of the manufacturing process.
Maintenance managers started using computerized maintenance management systems (CMMS) to track equipment history and schedule maintenance activities. German manufacturers, particularly in the automotive sector, pioneered the integration of robotics with digital control systems, setting early standards for automated production lines.
The Internet Era and Industry 3.0 (1990s-2000s)
The advent of the Internet transformed manufacturing capabilities. Companies like Rockwell Automation began developing ethernet-enabled industrial controllers, which allowed remote equipment monitoring for the first time.
Maintenance teams could access machine data from central control rooms rather than walking the factory floor.
General Electric implemented its first remote monitoring systems for industrial turbines during this period, marking the beginning of condition-based maintenance practices.
The Birth of Industry 4.0 (2011-Present)
The term “Industry 4.0”, sometimes called the fourth industrial revolution, was introduced at the Hannover Fair in 2011, marking the official beginning of the smart manufacturing era.
The German government’s High-Tech Strategy 2020 initiative formalized many concepts that would become central to smart manufacturing. This period saw the convergence of several technologies:
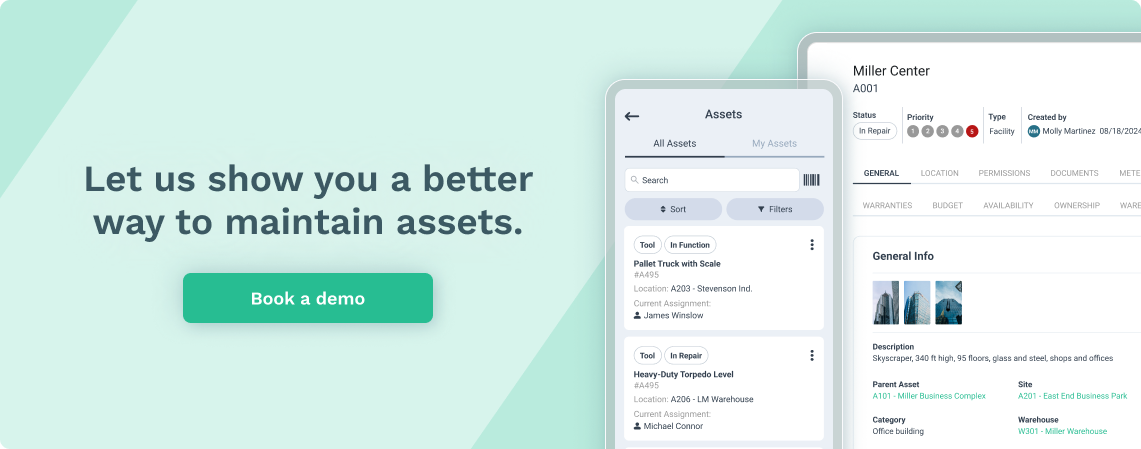
- Industrial Internet of Things (IIoT)
- Cloud Computing
- Advanced Analytics
- Artificial Intelligence
- Digital Twins
Corporate Adoption and Implementation
Early Adopters (2011-2015)
Siemens led the way with its Digital Enterprise platform, implementing comprehensive digital solutions in its factories first.
Its Amberg Electronics Plant became one of the first truly smart factories, with automated systems independently handling 75% of the value chain.
Boeing began implementing IoT sensors in its assembly lines, starting with its 787 Dreamliner production facility.
Mainstream Adoption (2015-2020)
As technology costs decreased and success stories emerged, mid-sized manufacturers began adopting smart manufacturing principles. Harley-Davidson’s York facility underwent a complete digital transformation, reducing its build-to-order cycle from 21 days to 6 hours.
Tesla built their Gigafactory from the ground up as a smart facility, incorporating lessons learned from traditional automotive manufacturers.
Recent Developments (2020-Present)
The COVID-19 pandemic accelerated smart manufacturing adoption as companies sought to improve resilience and remote monitoring capabilities.
Manufacturers like Procter & Gamble rapidly expanded their use of digital tools and remote maintenance capabilities.
Asian manufacturers, particularly in South Korea and Japan, made significant investments in smart manufacturing to maintain competitiveness.
Key Milestones in Maintenance Evolution
- 1968: Introduction of PLCs by GM
- 1980: First CMMS systems deployed
- 1995: Introduction of early predictive maintenance systems
- 2003: First widespread use of wireless sensors in manufacturing
- 2011: Launch of Industry 4.0 initiative
- 2015: Implementation of first large-scale digital twin systems
- 2018: Integration of AI-driven predictive maintenance
- 2020: Widespread adoption of remote monitoring and maintenance systems
Regional Adoption Patterns
Europe
Led by German manufacturers, European companies focused on comprehensive digital integration and standardization. The EU’s Factories of the Future initiative provided funding and a framework for smart manufacturing adoption.
North America
U.S. manufacturers typically took a more pragmatic approach, implementing smart technologies gradually based on clear ROI calculations. The Industrial Internet Consortium helped establish standards and best practices.
Asia
Japanese manufacturers emphasized integration with existing lean manufacturing systems. Chinese manufacturers made massive investments in smart manufacturing as part of the “Made in China 2025” initiative.
Impact on Maintenance Practices
The evolution of smart manufacturing has transformed maintenance from a cost center to a strategic function.
Modern maintenance managers work with data scientists and IT specialists to optimize equipment performance. Predictive maintenance has become the norm in advanced facilities, with some reporting up to 90% accuracy in failure prediction.
Learning from Historical Implementation
The history of smart manufacturing shows that successful adoption requires:
- Long-term strategic planning
- Significant investment in infrastructure and training
- Strong change management processes
- Integration with existing systems and processes
- Focus on people and skills development alongside technology
Understanding this historical context helps maintenance managers appreciate the evolutionary nature of smart manufacturing and more effectively plan their own digital transformation journeys.
Let me add a comprehensive analysis of the benefits and drawbacks of smart manufacturing, focusing on real-world implications:
Benefits and Drawbacks of Smart Manufacturing
Benefits
Enhanced Operational Efficiency
Smart manufacturing systems dramatically improve operational efficiency through continuous monitoring and optimization. For instance, Schneider Electric’s smart factory in Lexington, Kentucky, reported a 20% reduction in mean time to repair (MTTR) and a 90% improvement in predictive maintenance success rate. This efficiency level comes from the system’s ability to detect and address issues before they cause significant downtime.
Data-Driven Decision Making
Maintenance managers can make decisions based on real-time, accurate data rather than intuition or fixed schedules. A
t Intel’s semiconductor fabrication plants, data analytics have improved equipment effectiveness by 25% by identifying optimal maintenance windows and predicting potential failures with unprecedented accuracy. This data-driven approach ensures resources are allocated where they’re most needed.
Cost Reduction
Smart manufacturing significantly reduces operational costs across multiple areas. Procter & Gamble implemented smart manufacturing technologies across their facilities and reported a 20% reduction in maintenance costs and a 50% decrease in unplanned downtime.
These savings come from reduced energy consumption, optimized resource utilization, and prevented equipment failures.
Quality Improvement
Automated quality control systems in smart manufacturing environments can detect and correct quality issues in real time.
Toyota’s smart manufacturing facilities have reported a 60% reduction in quality defects after implementing AI-powered quality control systems that detect microscopic variations in product specifications.
Increased Safety
Smart manufacturing systems enhance workplace safety by automating dangerous tasks and providing early warning of potential hazards.
Mercedes-Benz’s smart factory in Sindelfingen, Germany, reported a 50% reduction in workplace incidents after implementing smart safety systems that use sensors and AI to monitor workplace conditions.
Drawbacks
High Initial Investment
Implementing smart manufacturing systems requires substantial upfront investment. A medium-sized manufacturing facility must invest between $5 million and $15 million to implement comprehensive smart manufacturing solutions.
This includes hardware, software, infrastructure upgrades, and staff training costs. Small and medium-sized enterprises often struggle to justify these initial costs.
Cybersecurity Risks
Connected systems create new vulnerabilities to cyber-attacks. In 2017, a major automotive manufacturer experienced a ransomware attack that shut down production for several days, resulting in millions of dollars in losses.
The interconnected nature of smart manufacturing systems means that a security breach in one area can affect the entire operation.
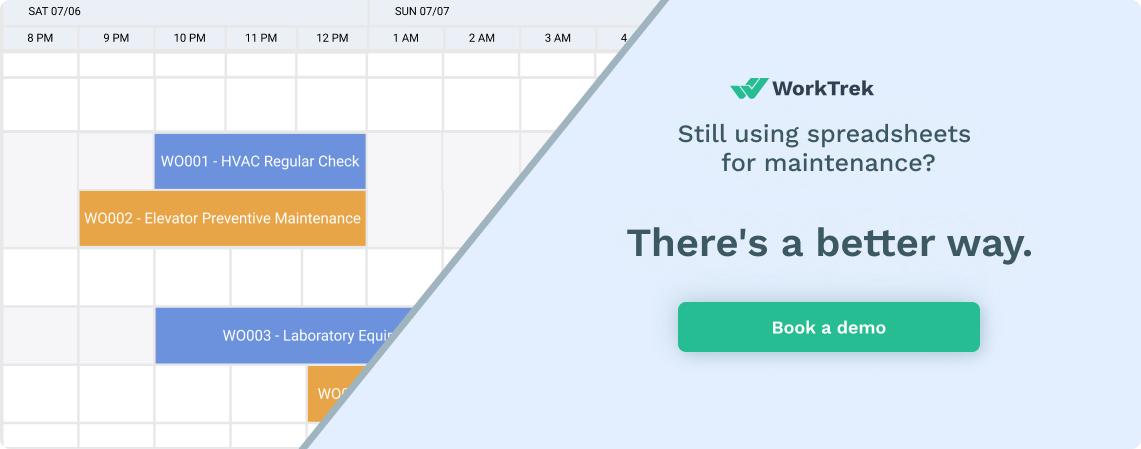
Technical Complexity
Smart manufacturing systems are highly complex and require specialized expertise to maintain and operate.
A Deloitte survey found that 60% of manufacturers struggle to find qualified personnel to manage their smart manufacturing systems. This complexity can lead to increased dependency on external vendors and consultants.
Integration Challenges
Legacy equipment and systems often pose significant integration challenges for the manufacturing industry. A pharmaceutical manufacturer spent three years and twice their initial budget to integrate their legacy equipment with new smart manufacturing systems. Some older equipment may need to be completely replaced, adding to the overall cost.
Data Management Challenges
Smart manufacturing systems generate massive amounts of data that must be stored, processed, and analyzed effectively.
A typical mass-production smart factory can generate several terabytes of data daily. Managing this data requires significant computing infrastructure and expertise in data analytics.
Resistance to Change
Human factors often present significant challenges in implementing smart manufacturing systems. A McKinsey study found that 70% of digital transformation projects in manufacturing fail due to employee resistance and inadequate change management.
Workers may fear job displacement or struggle to adapt to new technologies.
Dependency on Technology
Over-reliance on automated systems can create vulnerabilities when systems fail. This type of dependence can cause huge losses for organizations when critical equipment goes down.
This highlights the importance of having robust backup systems and manual override capabilities.
Training and Skill Development Requirements
Continuous training and skill development are necessary to keep pace with evolving technology. Companies typically need to spend 5-10% of their smart manufacturing budget on these activities, representing a significant ongoing cost and operational challenge.
Risk of Obsolescence
Rapid technological advancement means that smart manufacturing systems can quickly become outdated. Companies must continually invest in upgrades and updates to maintain their competitive advantage, which creates constant pressure to reinvest and upgrade systems.
Get a Free WorkTrek Demo
Let's show you how WorkTrek can help you optimize your maintenance operation.
Try for free