Get a Free WorkTrek Demo
Let's show you how WorkTrek can help you optimize your maintenance operation.
Try for freeBusinesses want to keep their equipment running smoothly. Predictive maintenance has emerged as a game-changing approach that uses data and smart technology to spot problems before they cause breakdowns.
This proactive strategy helps companies save money, reduce downtime, and extend equipment life by detecting potential failures before they happen.
Predictive maintenance is making waves in diverse industries, from factory floors to hospital equipment.
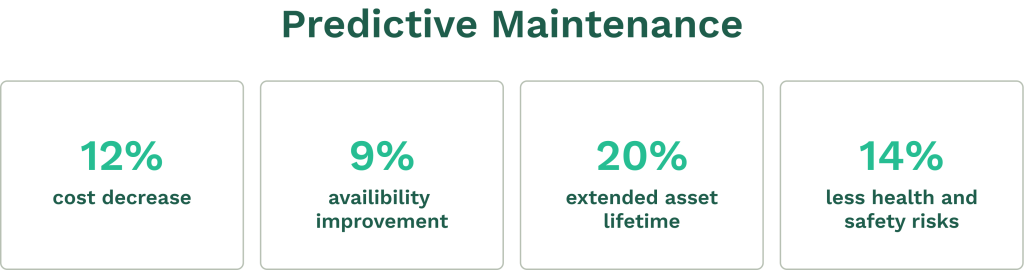
Manufacturing plants use vibration sensors on motors to predict failures, while energy companies monitor wind turbines remotely to schedule repairs during low-wind periods.
In aviation, aircraft engines have sensors that continuously analyze performance data, helping maintenance teams replace parts before they fail during flights.
Key Takeaways
- Predictive maintenance solutions use advanced analytics and sensor technology to identify equipment issues before failures occur, reducing unexpected downtime by up to 50%.
- Different industries implement unique predictive maintenance approaches based on their specific equipment needs, environmental conditions, and operational requirements.
- Integrating AI, machine learning, and a computerized maintenance management system (CMMS) like WorkTrek with predictive maintenance systems transforms maintenance from a cost center to a strategic business advantage across multiple sectors.
The Concept of Predictive Maintenance
Predictive maintenance transforms companies’ equipment health management through data-driven insights and timely interventions. It represents a shift from reactive fixes to proactive care that saves money and extends machine life.
Definition and Principles
Predictive maintenance is a strategy for proactively detecting potential defects and equipment anomalies before they cause failures. This can improve business operations and reduce operational costs.
Unlike preventive maintenance which follows fixed schedules, predictive maintenance relies on real-time data and condition monitoring.
The core principle involves using sensors and monitoring tools to track equipment performance metrics, such as vibration, temperature, noise levels, and oil conditions.
Modern predictive maintenance systems employ:
- Continuous monitoring of operational parameters
- Data analytics to identify patterns indicating potential failures
- Machine learning algorithms that improve predictions over time
- IoT connectivity for real-time insights
Techniques like infrared thermography, acoustic analysis, and vibration analysis help maintenance teams catch problems early when they’re cheaper to fix.
Benefits for Industries
Implementing predictive maintenance can deliver substantial benefits across diverse industrial settings, such as the oil and gas industry, among many others.
The most immediate benefit is significant cost reduction for critical assets through preventing catastrophic failures and extending equipment lifespan.
Companies experience fewer unexpected breakdowns, resulting in higher productivity and operational efficiency.
A well-implemented system can reduce downtime by 30-50% and extend machine life by 20-40%.
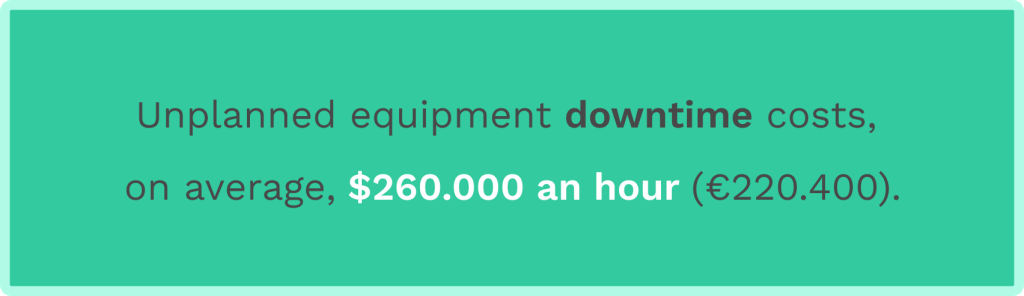
Other key benefits include:
- Improved safety conditions for workers
- Optimized maintenance scheduling based on actual equipment condition
- Reduced spare parts inventory costs
- Enhanced product quality through consistently functioning equipment
- Lower energy consumption from well-maintained systems
Predictive maintenance also supports sustainability initiatives by reducing waste and energy use. Organizations can make data-driven decisions about repairs versus replacements, optimizing resource allocation.
Predictive Maintenance in Manufacturing
Manufacturing facilities rely heavily on predictive maintenance to prevent costly downtime and ensure product quality.
These preventive maintenance programs utilize sensors, data analysis, and machine learning to detect potential issues before they cause breakdowns.
Equipment Failure Prediction
In manufacturing environments, predictive maintenance helps detect equipment issues before they lead to catastrophic failures. Sensors monitor key parameters like vibration, temperature, and acoustics to identify abnormal patterns. When these patterns emerge, maintenance teams receive alerts to address problems before equipment fails.
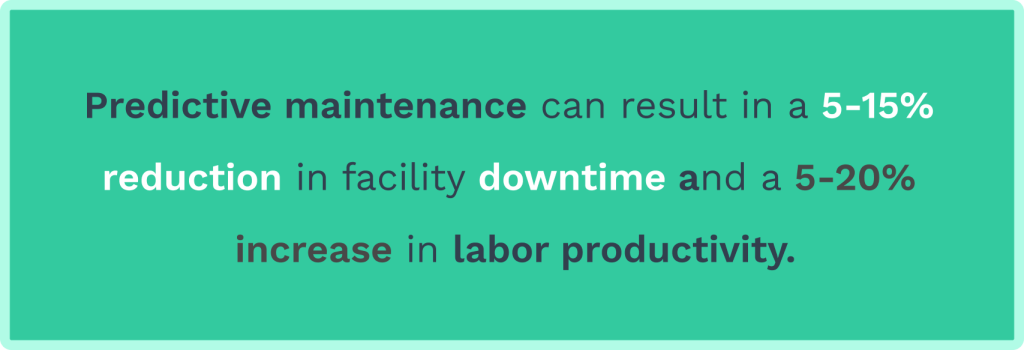
For example, a paper mill might use vibration analysis to detect bearing wear in critical motors. The system identifies subtle changes in vibration signatures that humans cannot perceive.
Machine learning algorithms continuously improve predictions by analyzing historical failure data. This creates a self-improving system that becomes more accurate over time.
Common monitoring parameters include:
- Vibration analysis
- Thermal imaging
- Oil analysis
- Acoustic monitoring
- Power consumption patterns
Quality Assurance Measures
A predictive maintenance program directly impacts product quality by ensuring that machines operate within optimal parameters.
Quality issues often appear when equipment moves from ideal settings before complete failure occurs.
Conveyor belt monitoring systems use vibration sensors to detect misalignments that could damage products. Similarly, precision manufacturing equipment utilizes real-time tolerance monitoring to predict when calibration will be needed.
Some advanced systems correlate maintenance data with quality control metrics. This allows manufacturers to identify which maintenance issues most significantly impact product quality.
Automated inspection systems also contribute to predictive maintenance by identifying patterns in defects that suggest equipment problems.
Case Study: Automotive Industry
With remarkable results, an automotive assembly plant implemented predictive maintenance across its welding robots. The system monitored electrical current signatures to detect when welding tips began to wear.
Before implementation, the plant averaged 4.7 hours of unplanned downtime per week. After deploying predictive maintenance, this dropped to just 0.8 hours—an 83% reduction.
Maintenance costs decreased by 47%, while product quality improved by 23%.
Wind energy companies use similar approaches by combining vibration analysis, oil testing, and thermal imaging to prevent turbine failures. These integrated systems allow for precise maintenance scheduling only when needed.
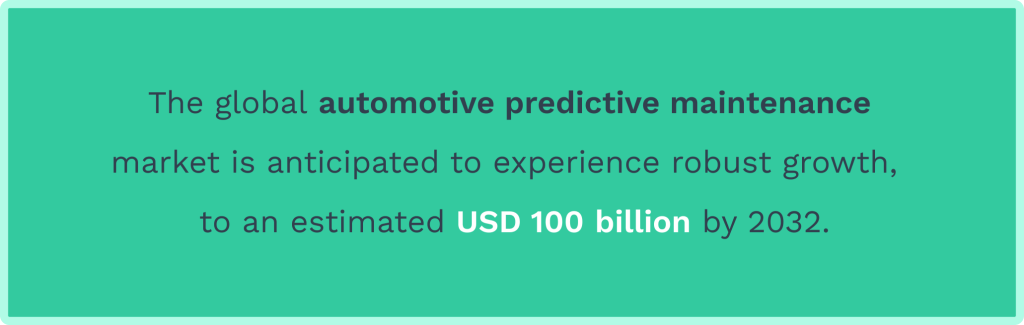
The automotive industry has been particularly successful with predictive maintenance because of its high production volumes and the significant cost of downtime. Many manufacturers report ROI within 6-12 months of implementation.
Use Cases in the Energy Sector
The energy sector has embraced predictive maintenance to enhance operational efficiency and sustainability.
These technologies help detect equipment failures before they occur, reducing downtime and maintenance costs while extending asset lifespans.
Wind Turbine Optimization
Wind energy companies utilize multiple predictive maintenance techniques to maximize turbine performance. Vibration analysis, oil analysis, and thermal imaging work together to identify potential issues before they cause shutdowns.
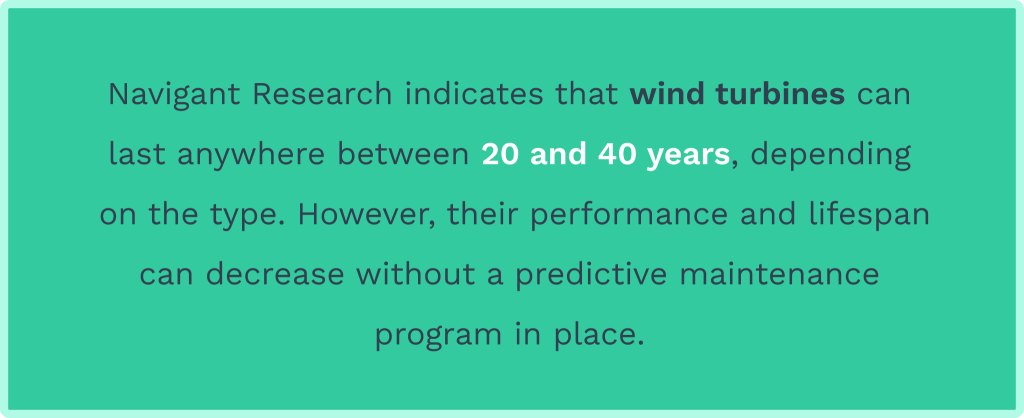
Sensors placed strategically on turbine components monitor abnormal vibrations that might indicate bearing failures or blade imbalances. This real-time data allows maintenance teams to schedule repairs during low-wind periods, minimizing production losses.
Weather pattern analysis integrates with maintenance schedules to optimize service timing. When high winds are forecasted, technicians can prioritize urgent repairs before storms arrive.
Remote monitoring systems reduce the need for dangerous climb-ups, improving worker safety. Drones equipped with cameras can inspect blade surfaces for cracks or erosion without stopping turbine operation.
Oil and Gas Equipment Surveillancework
The Oil and Gas industry is a $4 trillion industry. Oil and gas companies have extensively deployed predictive maintenance to prevent catastrophic failures and environmental incidents. Due to unplanned downtime, oil and gas companies lose $149 million annually.
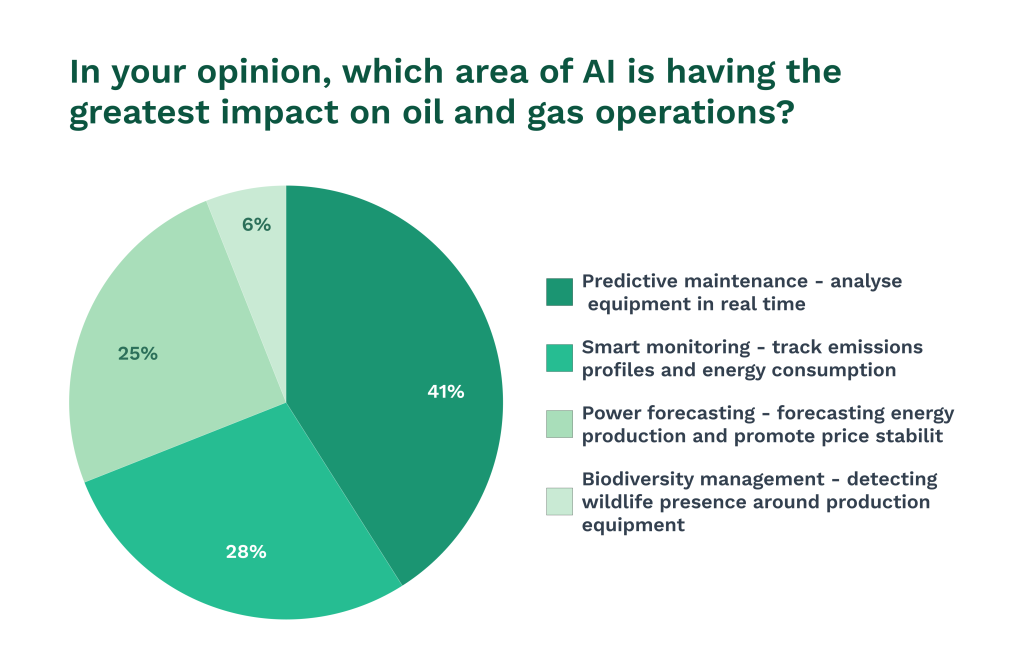
Given the size and complexity of the oil and gas industry, a single hour of downtime can cost around $500K. Therefore, they are highly motivated to reduce downtime.
Liquid leakage detection systems monitor pipelines and storage tanks for early signs of deterioration.
Acoustic sensors detect minute changes in equipment sounds that human ears cannot perceive. These subtle variations often indicate worn components or impending failures in pumps, compressors, and valves.
AI-powered systems analyze operational data from multiple sources to predict equipment failures up to weeks in advance. This allows companies to order parts and schedule maintenance during planned shutdowns.
Thermal imaging cameras identify hotspots in electrical systems and mechanical components. Overheating often precedes complete failures, making temperature monitoring critical for preventive action.
Corrosion monitoring tools use specialized sensors to track metal degradation in harsh environments, extending the life of expensive infrastructure.
Aviation Industry Applications
The aviation sector has emerged as one of the leading adopters of predictive maintenance technologies.
Airlines and aerospace companies implement advanced analytics to monitor aircraft components and enhance safety measures through data-driven approaches.
Aircraft Maintenance Scheduling
Airlines using predictive maintenance reap significant benefits by reducing unscheduled aircraft removals and increasing operational time. Modern aircraft generate terabytes of sensor data during each flight, providing valuable insights for maintenance teams.
Engine health monitoring systems track parameters like temperature, pressure, and vibration patterns to detect potential failures weeks before they occur. This early detection capability allows maintenance teams to schedule repairs during planned downtime.
Components like landing gear, hydraulic systems, and avionics undergo continuous monitoring through integrated sensor networks.
When anomalies appear, AI algorithms assess the severity and recommend appropriate maintenance actions.
Key benefits for airlines include:
- 30-40% reduction in maintenance-related delays
- Up to 20% decrease in repair costs
- Extended component lifespan through optimized servicing intervals
- Reduced inventory costs for spare parts
Flight Safety Enhancements
Predictive analytics revolutionizes aerospace maintenance by analyzing real-time sensor data to anticipate potential failures before they impact flight safety.
This proactive approach transforms how airlines manage risk.
Advanced algorithms can detect subtle changes in aircraft performance that might indicate developing problems. For example, slight variations in fuel consumption patterns might signal early-stage engine issues requiring inspection.
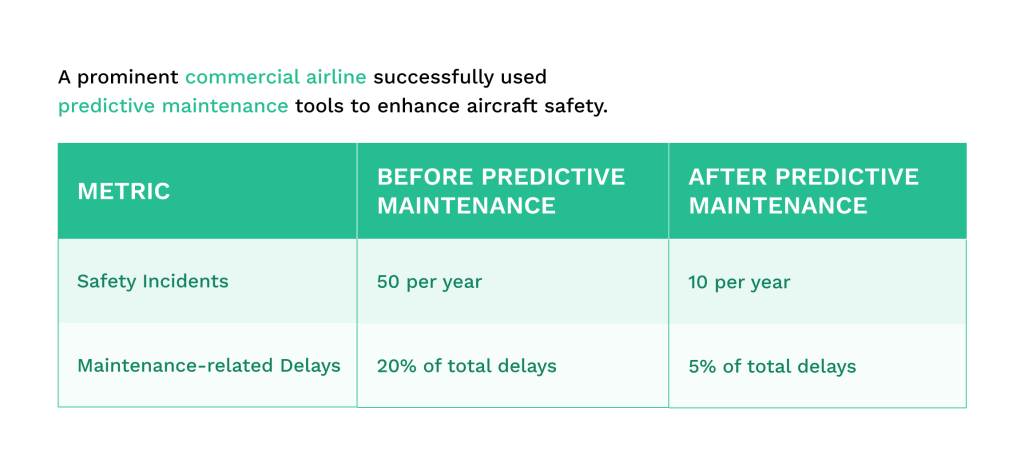
Weather pattern analysis combined with aircraft condition monitoring helps pilots and dispatchers make informed decisions about flight routes and altitudes. Aircraft maintenance relies on this approach to reduce wear on components while enhancing passenger comfort.
Safety improvements through predictive maintenance:
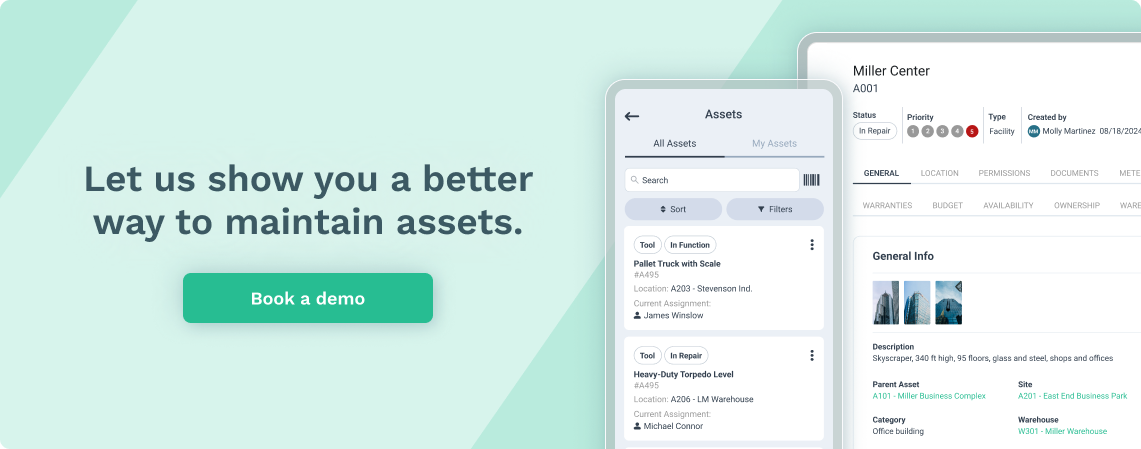
- Earlier detection of critical system degradation
- Reduced in-flight incidents related to mechanical failures
- Enhanced decision-making for flight crews
- More comprehensive documentation of aircraft health history
Automated damage recognition systems use computer vision to inspect aircraft surfaces during routine ground checks, identifying potential structural issues invisible to the human eye.
Predictive Maintenance in Transportation
Transportation systems depend heavily on reliable equipment and vehicles.
Predictive maintenance technologies have revolutionized monitoring and maintaining these assets, significantly reducing downtime and extending useful life.
Railway System Analytics
Railway systems use sophisticated predictive maintenance techniques to monitor critical components like wheels, tracks, and signals.
Vibration sensors on tracks detect subtle changes in train wheel patterns that might indicate early wear or damage.
Network Rail in the UK employs acoustic monitoring systems to identify failing bearings in passing trains. These systems can detect problems up to 3,000 miles before failure occurs, preventing dangerous derailments.
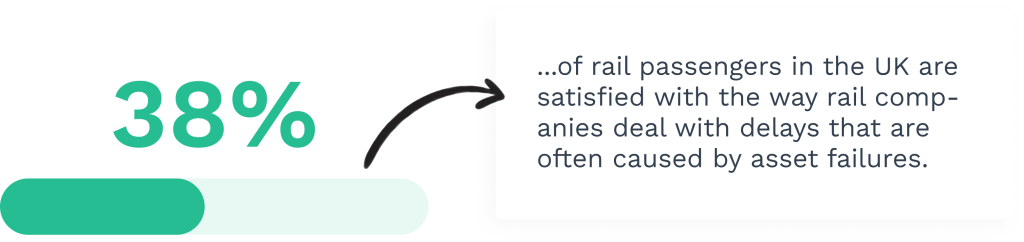
Temperature sensors along railway lines monitor track conditions to prevent buckling in extreme heat. This gives maintenance teams time to address issues before they affect service.
Deutsche Bahn uses AI algorithms to analyze data from multiple sensors, resulting in a 25% reduction in unplanned maintenance. Their system predicts when catenary wires and switches need servicing days before problems become visible.
Fleet Management Solutions
Commercial vehicle fleets implement predictive maintenance solutions to maximize vehicle uptime and operational efficiency. Onboard diagnostic systems continuously monitor engine performance, transmission behavior, and brake wear.
Toyota partnered with IBM’s Maximo solution to analyze real-time vehicle data. This system flags potential failures before they occur, allowing for scheduled maintenance that doesn’t disrupt delivery schedules.
Oil analysis in fleet vehicles detects microscopic metal particles that indicate internal engine wear. Maintenance teams can predict when specific components need replacement by tracking these particles over time.
Fleet managers use dashboards that display vehicle health scores based on multiple data points. These scores help prioritize maintenance activities and optimize resource allocation.
Battery health monitoring in electric delivery vehicles uses thermal imaging to identify cells degrading faster than others. This prevents unexpected failures during operation and extends overall battery life.
Smart Infrastructure and Buildings
Modern buildings now use predictive maintenance to prevent problems and save money. Smart systems collect sensor data to catch issues before they cause failures or expensive damage.
HVAC System Monitoring
HVAC systems benefit greatly from predictive maintenance techniques. Sensors measure temperature, airflow, pressure, and energy consumption to detect performance issues early. When a system shows unusual patterns, maintenance teams receive alerts.
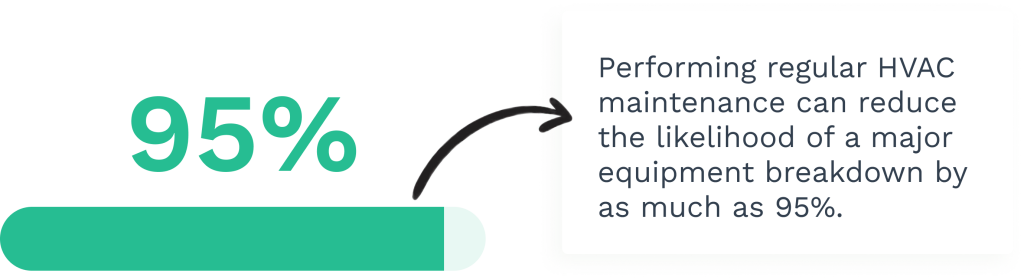
For example, algorithms can identify when an air handler works harder than normal, suggesting a potential filter clog or motor problem. This early warning prevents complete system failure during extreme weather conditions.
Key HVAC monitoring parameters include:
- Temperature differential across components
- Power consumption patterns
- Vibration analysis of moving parts
- Refrigerant pressure readings
Many smart buildings use IoT integration that lets technicians view HVAC performance remotely. This remote monitoring capability reduces inspection visits and helps prioritize maintenance work.
Structural Health Monitoring
Buildings face ongoing stress from weather, usage, and aging. Condition monitoring systems use sensors to track structural integrity over time.
These systems measure:
- Vibration patterns revealing potential weaknesses
- Settlement and shifting of foundations
- Material fatigue in critical support elements
- Moisture levels that might lead to corrosion
Acoustic sensors detect subtle changes in how sound moves through structures. This technology can identify developing cracks or weakening joints before they become visible to inspectors.
Advanced buildings incorporate strain gauges on key structural elements. These devices measure tiny changes that might indicate developing problems with load-bearing components.
These monitoring systems quickly assess building safety after earthquakes or severe storms. This helps reduce costly inspections and provides data-driven decisions about occupancy.
Healthcare Industry Implementation
Predictive maintenance in healthcare delivers significant benefits through equipment reliability and enhanced patient outcomes.
Healthcare facilities have started leveraging data analytics and sensor technology to prevent failures and optimize care delivery.
Medical Equipment Upkeep
Hospital equipment is critical, and failure can be life-threatening. This makes predictive maintenance paramount.
Modern healthcare facilities have implemented vibration analysis and acoustic monitoring to detect early signs of deterioration in critical equipment.
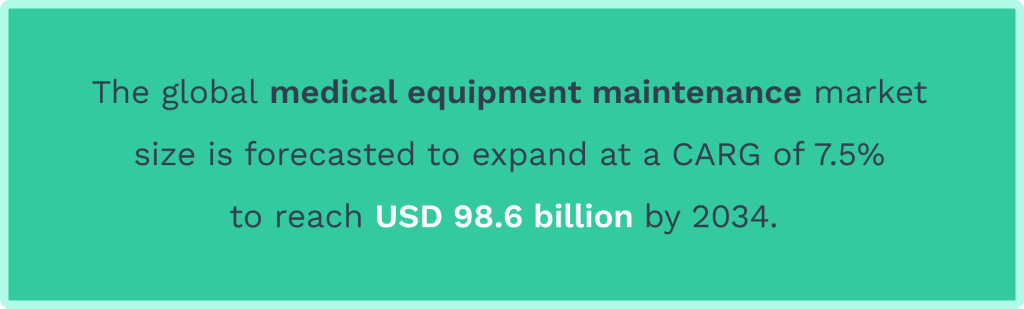
One example is that predictive maintenance greatly benefits MRI machines. Sensors track helium levels, cooling system performance, and magnetic field stability to prevent unexpected downtime.
Hitachi’s Sentinel Analytics, a predictive maintenance system for MRI machines, has been shown to reduce downtime by 16.3% compared to before its implementation. This reduction in downtime is particularly significant for superconducting MRI systems.
GE Healthcare’s OnWatch Predict for MRI has shown even more impressive results, reducing unplanned downtime by up to 40% and cutting customer-initiated service requests (often sources of stress for hospital imaging staff) by as much as 35%.
Financial Implications
The financial implications are substantial. A typical medium-sized healthcare facility may allocate approximately $5 million annually for medical equipment maintenance, while an average healthcare system can incur annual costs of around $50 million. Full-service maintenance for a single CT or MRI machine can cost over $100,000 annually.
Patient Monitoring Systems
Patient monitoring systems also receive predictive care through continuous performance tracking. Algorithms detect subtle changes in measurement accuracy, power fluctuations, and component wear. Healthcare providers save millions annually by preventing emergency repairs and avoiding patient care disruptions.
Predictive maintenance software integrates with hospital management systems to schedule maintenance during low-usage periods. This integration minimizes disruption to patient care while maximizing equipment availability.
Patient Care Improvement
Beyond equipment reliability, predictive maintenance improves patient outcomes through data-driven interventions. Smart beds with embedded sensors monitor patient movement patterns and predict potential pressure ulcer development sites.
Remote patient monitoring devices employ predictive algorithms to identify potential failures before they affect patient care. These systems analyze battery life, connectivity patterns, and sensor accuracy to ensure reliable monitoring of vital signs.
Medication Dispensing Systems
Predictive maintenance, which involves continuous analysis of mechanical components, benefits medication-dispensing systems. Early wear detection in dispensing mechanisms prevents medication errors and ensures accurate dosing.
Predictive analytics extends to patient flow management, identifying potential bottlenecks in care delivery.
This approach optimizes resource allocation, reduces wait times, and improves overall patient satisfaction while maximizing equipment utilization.
Predictive Maintenance Technologies
Modern predictive maintenance relies on advanced technologies that collect, analyze, and act on equipment data. These systems work together to help companies prevent breakdowns and extend machine lifespans.
Internet of Things (IoT) Devices
IoT devices form the foundation of effective predictive maintenance by continuously monitoring equipment health. These smart sensors attach directly to machinery and measure critical parameters like temperature, vibration, pressure, and acoustics in real time.
For example, vibration analysis sensors can detect subtle changes in equipment operation that humans cannot perceive. When a motor bearing begins to wear, these sensors identify the issue weeks before failure occurs.
Modern IoT devices are becoming increasingly affordable and compact. Many now operate wirelessly and can be powered by batteries or energy harvesting techniques, making installation possible even on older equipment.
The data captured by these sensors creates a continuous stream of information that feeds into centralized systems. This allows maintenance teams to monitor hundreds of assets simultaneously without physical inspection.
Machine Learning and Data Analytics
Machine learning algorithms transform raw sensor data into actionable maintenance insights. These AI systems identify patterns and anomalies that would be impossible for humans to detect manually.
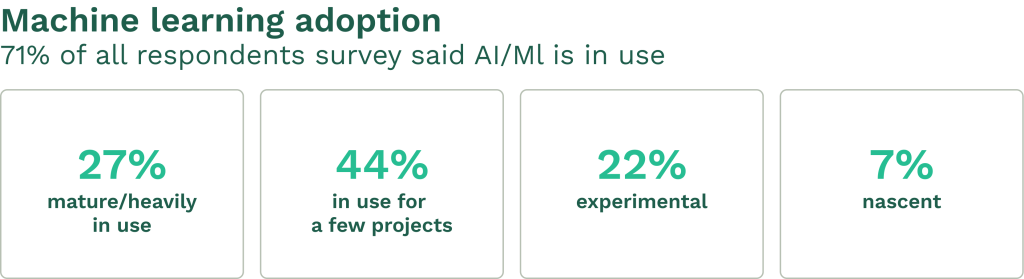
AI-powered predictive maintenance helps hospitals extend equipment lifespans, reduce costly failures, and ensure reliable patient care. By automating monitoring and alerts, hospital staff can focus more on patient care, boosting productivity and enhancing service quality.
After collecting sufficient historical data, predictive models can determine when specific components will likely fail. For instance, algorithms can analyze oil samples to predict engine component wear accurately.
Several industries leverage these capabilities:
- Manufacturing: Detecting tool wear before product quality suffers
- Energy: Predicting generator failures to prevent outages
- Transportation: Forecasting vehicle component failures to optimize maintenance schedules
The most advanced predictive maintenance companies use AI to move beyond simple failure prediction. Their systems can recommend optimal repair times, estimate remaining useful life, and suggest specific maintenance procedures.
Remote Monitoring Software
Remote monitoring platforms serve as the control center for predictive maintenance operations. These software solutions aggregate data from multiple sources and present it through intuitive dashboards.
Maintenance teams can access equipment status from anywhere using web or mobile interfaces. Critical alerts, often sent through smartphone notifications, notify technicians immediately when systems detect potential problems.
These platforms typically include:
- Real-time equipment status visualization
- Historical performance trending
- Automated work order generation
- Maintenance schedule optimization
- Parts inventory management
Many industries benefit from remote monitoring capabilities. Food processing companies use these systems to ensure production equipment meets strict hygiene standards, while mining operations monitor equipment across vast geographical areas.
Advanced platforms integrate with enterprise resource planning (ERP) systems to streamline maintenance workflows and provide a cost analysis of maintenance activities.
Computerized maintenance management system
Computerized Maintenance Management Systems (CMMS) have revolutionized how organizations approach equipment maintenance by enabling the shift from reactive to predictive maintenance strategies. This transformation is delivering substantial benefits across multiple industries.
CMMS platforms collect real-time data from machinery sensors in manufacturing to identify patterns that precede failures. By analyzing vibration patterns, temperature fluctuations, and output quality metrics, these systems can alert maintenance teams before catastrophic breakdowns occur. This predictive approach reduces unplanned downtime by up to 50% and extends equipment lifespan by 20-40%.
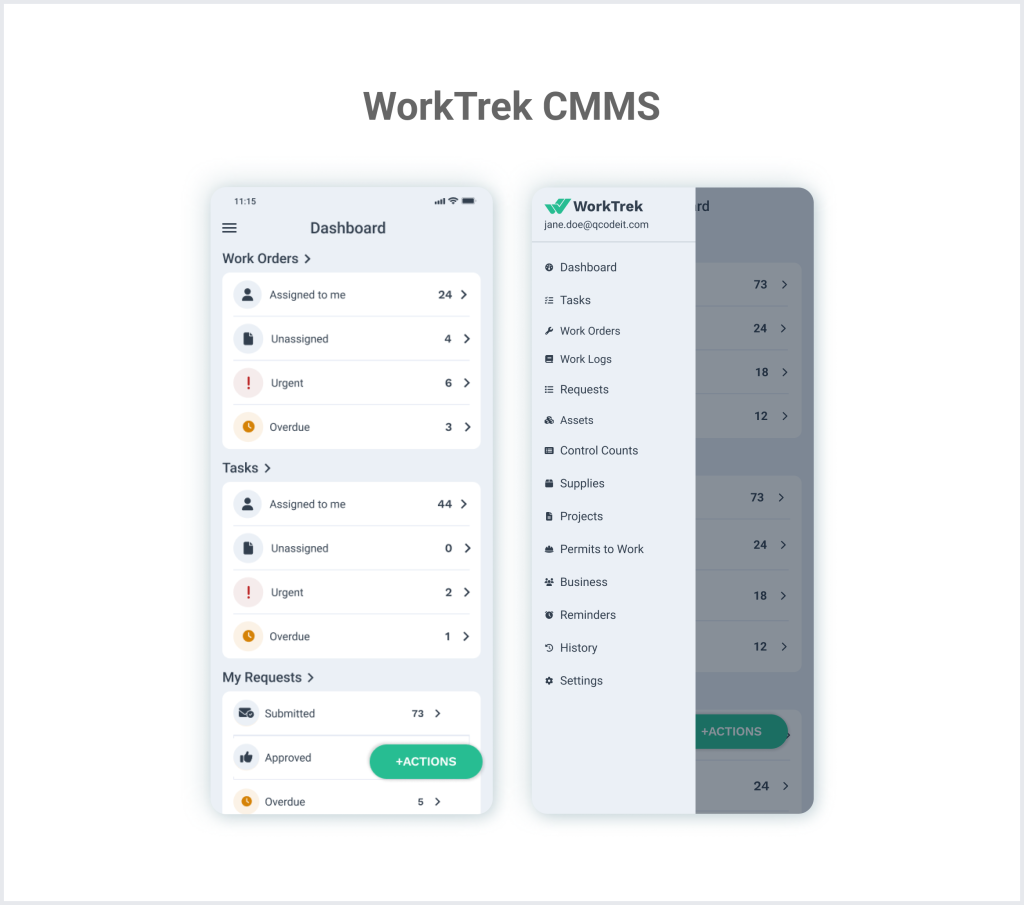
Healthcare facilities leverage CMMS to ensure critical equipment like MRI machines, ventilators, and laboratory analyzers remain operational.
The predictive capabilities help schedule maintenance during non-peak hours, minimizing disruption to patient care while ensuring regulatory compliance with precise documentation of all maintenance activities.
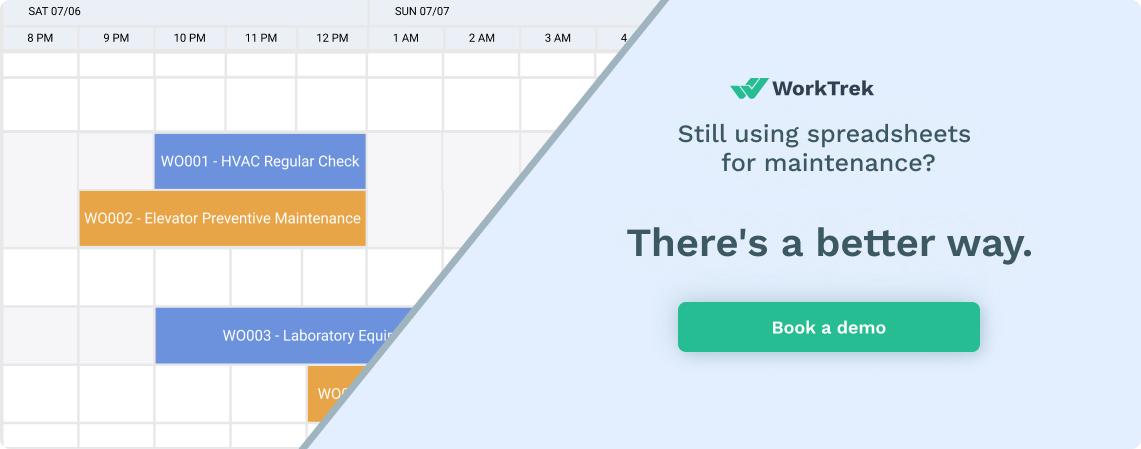
CMMS monitors power generation equipment, transmission infrastructure, and distribution networks in energy and utilities. Remote monitoring capabilities are particularly valuable for geographically dispersed assets like wind turbines or pipeline networks.
Companies avoid costly emergency repairs and maintain service reliability by predicting potential failures in these hard-to-reach locations.
Transportation and logistics operations use CMMS to predict maintenance needs for fleet vehicles, cargo-handling equipment, and infrastructure.
The systems analyze engine diagnostics, brake performance, and other critical systems to schedule maintenance before vehicles break down on delivery routes, preventing costly delays and service interruptions.
Facility management teams employ CMMS to maintain building systems, including HVAC, elevators, and security equipment. By integrating with CMMS, predictive maintenance ensures optimal building performance, energy efficiency, and occupant comfort.
The ROI of CMMS-powered predictive maintenance is compelling across all these sectors:
- Reduced maintenance costs
- Reduction in costly repairs
- Decreased equipment downtime,
- Extended asset management and performance
- Optimized inventory management for spare parts
- Improved resource allocation for maintenance personnel
- Improved asset performance
- Improved proactive maintenance planning
As IoT sensor technology advances and machine learning algorithms become more sophisticated, CMMS platforms will grow more powerful in their predictive capabilities.
Challenges and Considerations
While predictive maintenance offers substantial benefits, organizations face several hurdles when implementing these systems.
If not adequately addressed, technical, financial, and operational obstacles can impact successful deployment.
Data Privacy and Security
Data security remains one of the most significant challenges in implementing predictive maintenance. Connected sensors and IoT devices collect vast amounts of operational data that may contain sensitive information about production processes or intellectual property.
Organizations must establish robust data protection protocols to prevent unauthorized access or breaches. This includes encryption of data both at rest and in transit.
Compliance with regulations like GDPR or industry-specific requirements adds another layer of complexity. Companies operating globally must navigate varying regional data privacy laws.
Many maintenance teams struggle to determine who should access which data streams.
Proper authentication systems and access controls are essential for maintaining data integrity while still allowing the right personnel to view critical information.
Cost-Benefit Analysis
Predictive maintenance requires significant upfront investment in sensors, data infrastructure, analytics software, and staff training.
Organizations often face increasing maintenance costs initially before seeing returns.
The ROI calculation must account for:
- Hardware acquisition and installation costs
- Software licensing and customization expenses
- Training and change management resources
- Ongoing system maintenance requirements
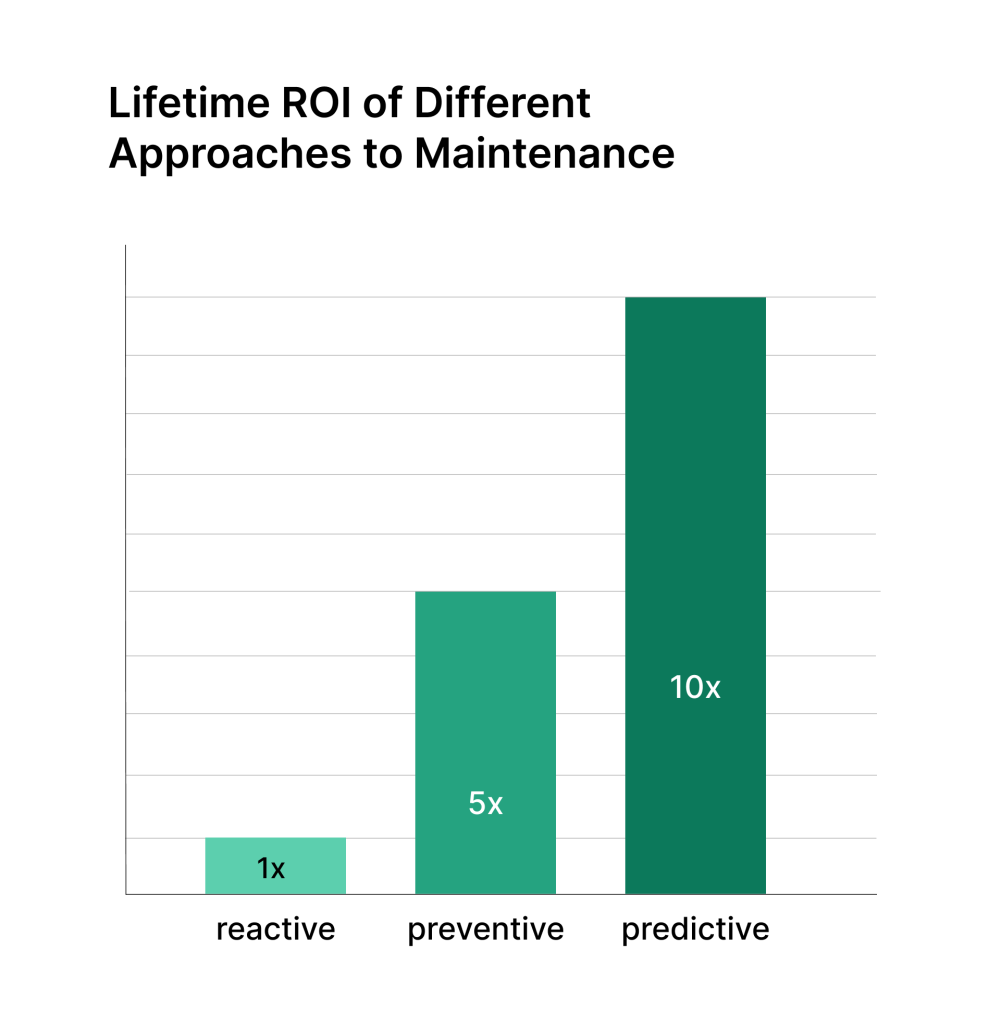
Many companies struggle to quantify the benefits, particularly indirect ones like improved product quality or extended equipment life. A comprehensive analysis should include tangible savings from reduced downtime and intangible benefits.
Small organizations may find the investment particularly challenging without clear proof of returns. Phased implementation approaches can help manage costs while incrementally demonstrating value.
Integration with Existing Systemswork
Incorporating predictive maintenance into legacy operational technology presents substantial technical hurdles.
Many facilities operate with equipment of varying ages and communication protocols not designed for modern connectivity.
Technological complexities arise when attempting to standardize data from diverse sources. Different machines may generate different data formats or measurement units, requiring normalization.
Integration often demands custom solutions rather than off-the-shelf products. Maintenance teams must collaborate closely with IT departments to ensure seamless data flow.
Resistance to change can impede adoption. Maintenance personnel may be reluctant to transition from familiar reactive approaches to data-driven methods. Comprehensive training programs and clear communication about the benefits help address this challenge.
Future Trends in Predictive Maintenance
Predictive maintenance tools and technologies are evolving rapidly, with significant innovations in AI capabilities and increased automation through robotics.
The predictive maintenance market is forecasted to reach USD 80.2 billion by 2034 at a 10.9% CAGR. The global predictive maintenance market was valued at USD 8.05 billion in 2023 and is expected to grow at a CAGR of 35.4% during the forecast period.
Advancements in AI and Predictive Analytics
The predictive maintenance market is experiencing substantial growth, reaching $5.5 billion in 2022 with a projected CAGR of 17% by 2028.
Integrating artificial intelligence (AI) promises to automate further and refine maintenance processes.
At the same time, advancements in diagnostics and prognostics are set to offer even more precise insights into equipment health. Analyzing sensor data, AI and ML algorithms enable more accurate predictions of equipment failures.
Machine Learning
Machine learning models are becoming increasingly accurate at predicting equipment failures days or weeks before they occur. These models integrate multiple data streams simultaneously, including vibration, temperature, acoustic, and operational data.
Digital Twins
Digital twins—virtual replicas of physical assets—are gaining prominence in predictive maintenance strategies. They simulate equipment performance under various conditions, allowing maintenance teams to test different scenarios without risking actual machinery.
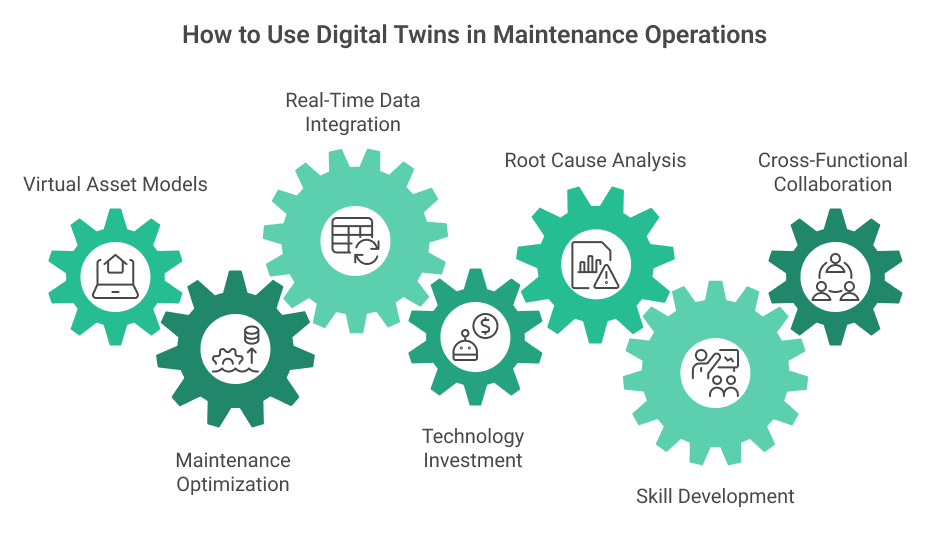
Edge computing reduces latency in predictive maintenance systems by processing data directly on devices rather than sending everything to the cloud. This enables real-time decision-making for critical equipment.
Growing Role of Robotics
Autonomous inspection robots are increasingly deployed in hazardous or difficult-to-access environments. These robots can safely perform routine inspections in areas like offshore oil platforms or chemical plants without endangering human workers.
Drone technology is integrated with predictive maintenance systems, particularly for inspecting large-scale infrastructure like power lines, wind turbines, and bridges. Equipped with thermal cameras and sensors, drones can detect anomalies invisible to the human eye.
Collaborative robots (cobots) work alongside maintenance technicians to enhance precision in repair tasks. These robots can handle repetitive diagnostic procedures while technicians focus on complex problem-solving.
Remote monitoring capabilities have expanded significantly. Robots can now autonomously conduct infrared thermography and acoustic analysis in facilities with minimal human supervision.
Conclusion
Predictive maintenance represents a revolutionary approach to equipment management across industries.
By leveraging advanced technologies like IoT sensors, AI algorithms, and remote monitoring systems, organizations can anticipate failures before they occur, dramatically reducing downtime and maintenance costs.
The applications are diverse and impactful, from manufacturing plants monitoring motor vibrations to airlines tracking aircraft engine performance. The benefits extend beyond cost savings, including improved safety, enhanced product quality, and extended equipment lifespan.
Predictive maintenance is poised for further growth as technologies evolve through AI advancements and robotics integration.
Despite challenges in data security, implementation costs, and system integration, the return on investment makes predictive maintenance increasingly attractive to forward-thinking companies.