What is Acoustic Analysis?
Have you ever wondered what’s behind your car’s strange sounds or how voice recognition systems work? Acoustic analysis holds the answers. This scientific process involves studying sound waves to understand their properties and behavior in different environments.
Acoustic analysis is the measurement and assessment of sound waves using specialized hardware and software to provide quantifiable information about the acoustic signal. It’s sometimes called noise and vibration analysis, as it examines audible sounds and vibrations that human ears might not detect.
Acoustic energy represents the intensity and frequency components of sounds, which are crucial for understanding the loudness and characteristics of different sound types.
This field has wide-ranging applications, from measuring equipment vibrations to analyzing voice patterns and frequency range.
Sound waves exist around us, from homes to industrial settings; acoustic analysis helps us understand them.
Companies use this technology to detect potential equipment failures before they happen, while healthcare professionals use it to diagnose voice disorders.
With advanced software tools now available, acoustic analysis has become more accessible and powerful than ever.
Fundamentals of Acoustic Analysis
Acoustic analysis examines sound waves and their properties to measure and understand voice and environmental sounds. These measurements help identify patterns, irregularities, and characteristics in sound that can be applied in multiple fields.
Sound Waves and Properties
Sound waves are pressure changes that travel through an elastic medium like air or water. These waves have key properties that acoustic analysis measures:
- Frequency: Measured in Hertz (Hz), determines pitch
- Amplitude: Relates to loudness or volume
- Wavelength: The distance between consecutive wave peaks
- Phase: Position of the wave relative to a reference point
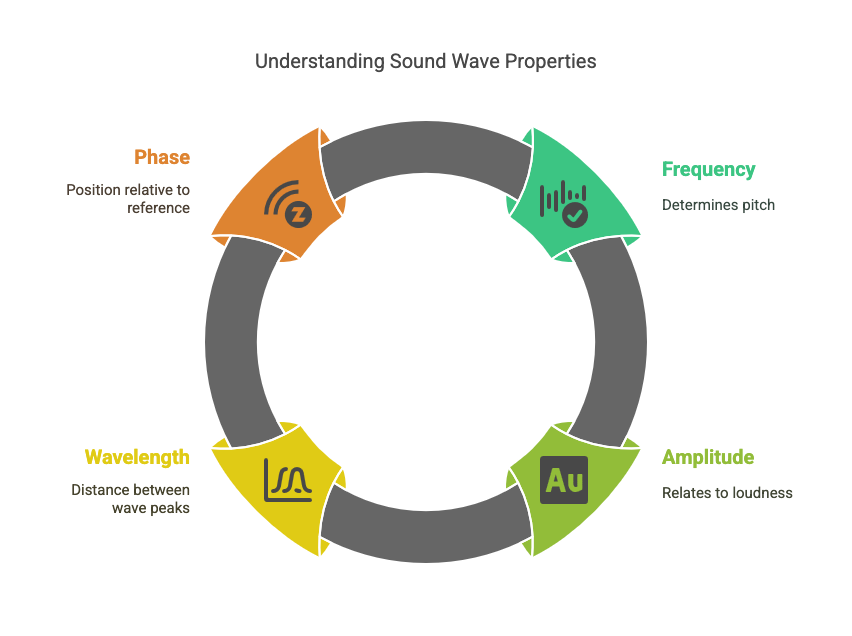
Source: WorkTrek.com
The fundamental frequency is the lowest in a sound wave and is a key measure in vocal studies and speech analysis.
Sound waves can be simple or complex, depending on their pattern. Simple waves have a single frequency, while complex waves contain multiple frequencies.
When analyzing sound, specialists examine how these waves interact with environments and materials. This includes studying reflection, refraction, diffraction, and absorption properties.
Acoustic Signal Characteristics
Acoustic signals have distinguishing characteristics that acoustic analysis measures and evaluates. These include:
Temporal features:
- Duration of sounds
- Rise and decay times
- Rhythmic patterns
Spectral features:
- Frequency distribution
- Harmonic structure
- Formants (resonant frequencies)
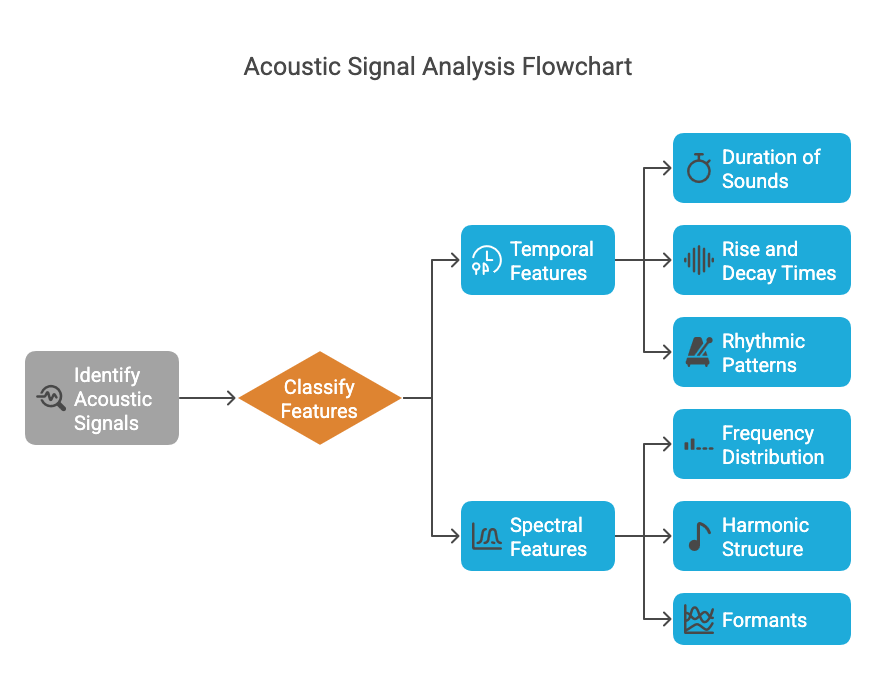
Source: WorkTrek.com
The lowest frequency component is crucial for determining the frequency of sounds like newborn cries and voiced speech.
Acoustic analysis tools transform sound waves into visual representations called spectrograms. These show how frequency components change over time, with color indicating intensity.
Regular vocal fold vibrations in voice analysis provide quantitative information about voice quality. Measurements like jitter (frequency variation) and shimmer (amplitude variation) help evaluate voice disorders.
For environmental or mechanical sounds, acoustic analysis detects abnormal patterns that might indicate equipment failures or structural issues.
Acoustic Analysis Techniques
Acoustic analysis uses several specialized techniques to measure and interpret sound data. These methods examine different aspects of acoustic signals to provide a complete picture of sound properties.
Spectrogram Analysis
Spectrogram analysis visually represents sound, showing how frequencies change over time. This technique displays a 3D view with time on the x-axis, frequency on the y-axis, and intensity shown through color variation.
Spectrograms are useful tools for identifying patterns in speech and music. They can reveal formants (resonant frequencies) in speech that help distinguish between vowel sounds.
Modern acoustic analysis software makes creating spectrograms simple. Engineers and scientists use them to:
- Detect mechanical faults through vibration patterns
- Analyze bird songs and animal vocalizations
- Study speech disorders by comparing normal and pathological patterns
The color intensity in spectrograms helps experts quickly identify important features that might be missed in other analysis methods.
Fourier Transform and Frequency Analysis
Fourier Transform converts time-domain signals into frequency-domain representations. This mathematical technique breaks complex sound waves into their component frequencies.
The Fast Fourier Transform (FFT) is the most common implementation in acoustic analysis software. It efficiently processes digital sound data to reveal frequency content.
Key applications include:
- Noise reduction in audio recordings
- Voice recognition systems
- Musical instrument analysis
Fourier Transform can help identify bearing faults in rotating machinery by revealing unique vibration patterns.
Engineers often use FFT to identify unwanted frequencies in mechanical systems. This helps pinpoint problems in rotating equipment before catastrophic failures occur.
Advanced analysis builds on basic FFT and includes techniques like power spectral density and cepstral analysis. These methods help extract more specific information from acoustic signals.
Waveform Analysis
Waveform analysis examines the raw amplitude of sound signals over time. This fundamental technique examines the sound wave’s shape to determine its essential properties.
Acoustic measurement tools capture these waveforms as graphs showing pressure variations. Analysts can identify essential features like:
- Amplitude: Indicating volume or intensity
- Period: Revealing frequency characteristics
- Attack and decay: Showing how sounds start and fade
Waveform analysis helps determine loudness levels in environmental noise monitoring. It’s also crucial for speech therapy, where timing patterns need careful assessment.
Music producers use waveform visualization to edit recordings precisely. They can see exactly where sounds begin and end, enabling precise edits.
Applications of Acoustic Analysis
Acoustic analysis techniques are employed across multiple fields to solve complex sound problems.
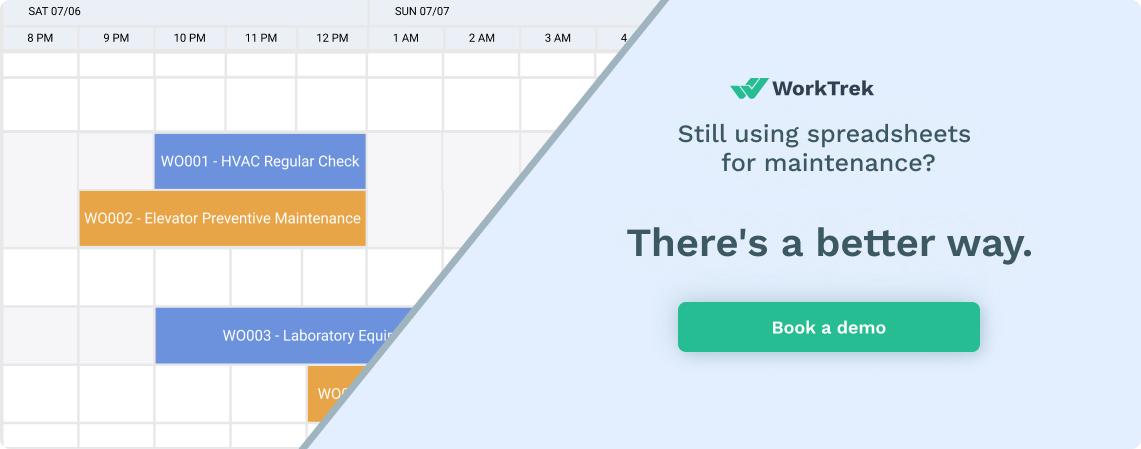
The technology helps professionals extract valuable information from audio signals for practical communication, entertainment, and environmental monitoring applications.
Speech Recognition
Speech recognition systems use acoustic analysis to convert spoken language into text. These systems analyze speech signals by extracting features like pitch, formants, and speech energy.
Vocal folds open and close to create periodic signals, essential for generating fundamental frequencies in speech.
Modern voice assistants like Siri, Alexa, and Google Assistant rely on acoustic analysis to understand spoken commands. The technology breaks down speech into phonemes and uses machine learning to match them to words.
In healthcare, acoustic analysis helps diagnose speech disorders by measuring voice parameters like jitter, shimmer, and harmonic-to-noise ratio. These measurements provide objective data for tracking treatment progress.
Voice biometric systems use unique vocal characteristics for security applications to verify a person’s identity. This technology is increasingly used in banking, customer service, and access control systems.
Music Information Retrieval
Music information retrieval (MIR) applies acoustic analysis to extract meaningful data from music recordings. This field helps develop music recommendation systems by analyzing tempo, rhythm, and tonal features. The shape and size of the vocal tract influence the characteristics of the sound waves produced, affecting both speech and singing.
Music streaming platforms use these techniques to classify songs by genre, mood, and similarity. The analysis identifies patterns in the audio signal that human listeners might recognize as “happy,” “energetic,” or “relaxing.”
Automatic music transcription converts recordings into musical notation through advanced acoustic analyses like pitch detection and onset detection. This helps musicians learn songs and researchers study musical structures.
Music production tools use acoustic analysis for automatic beat matching, pitch correction, and instrument separation. These technologies enable producers to manipulate recorded sounds in ways previously impossible.
Environmental Sound Analysis
Environmental sound analysis monitors and classifies sounds in natural and urban settings. This application helps scientists track wildlife populations by identifying animal calls without direct observation. Acoustic analysis helps monitor wildlife populations and identify noise pollution sources.
Urban noise monitoring systems use acoustic analysis to measure sound levels and identify noise pollution sources. Cities use this data to enforce noise regulations and plan infrastructure.
In industrial settings, sonic analysis detects machine faults by identifying changes in equipment sounds. Unusual acoustic patterns can indicate worn bearings or other mechanical problems before they cause failures.
Security systems employ acoustic analysis to detect and classify sounds like breaking glass or gunshots. These systems can automatically alert authorities when specific sound patterns are detected.
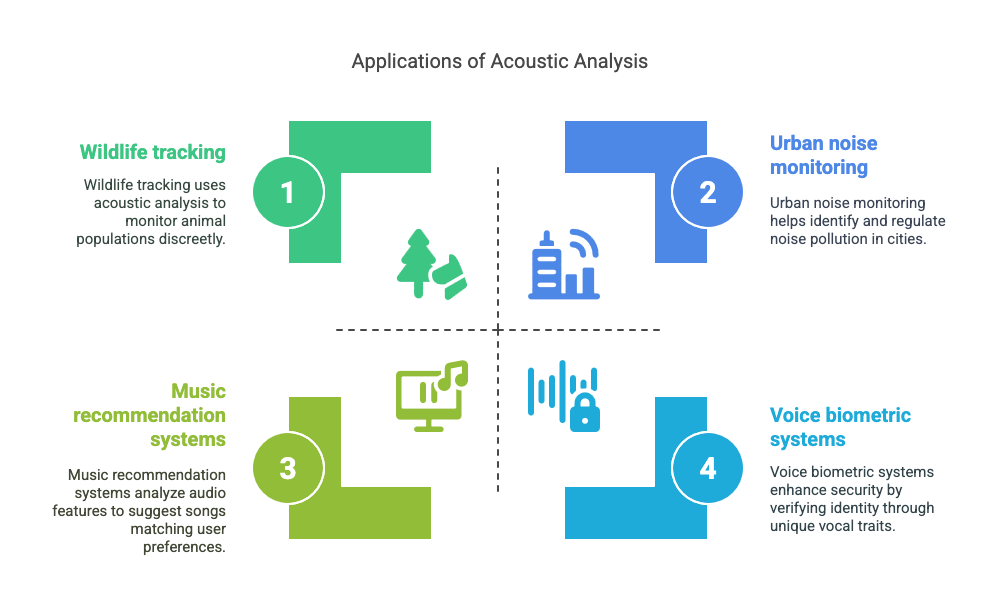
Source: WorkTrek.com
Acoustic Measurements: Tools and Equipment
Accurate acoustic analysis requires specialized equipment to capture, measure, and analyze sound waves. The right tools help professionals quantify frequency response, sound pressure levels, and other key acoustic parameters.
Key issues such as recording conditions, microphone type, and analytical software significantly impact the validity and reliability of acoustic analysis data.
Microphones and Recorders
Sound level meters are among the most common acoustic measurement devices. These handheld tools measure sound pressure levels and provide immediate readings in decibels.
Professional models often include frequency weighting filters (A, C, Z) to match human hearing perception.
Measurement microphones differ from regular recording mics. They feature flat frequency response curves and precise calibration for accurate data collection. Types include free-field, pressure, and random incidence microphones for specific measurement scenarios.
Acoustic measurement systems frequently incorporate multiple microphone arrays. These configurations allow sound source localization and mapping sound fields in complex environments.
Portable audio recorders with high-quality preamplifiers help capture acoustic data for later analysis. Many professionals use multichannel recorders to capture sound simultaneously from different positions within a space.
Software for Acoustic Analysis
Spectrum analyzers convert time-domain audio signals into frequency-domain data. These tools display the strength of different frequency components within a sound, revealing details invisible to the ear alone.
Real-time analyzers (RTAs) show frequency content as it occurs, making them valuable for live monitoring and adjustment. Most acoustic software includes octave and 1/3 octave analysis capabilities to match how humans perceive sound.
Room acoustic measurement software calculates important parameters like reverberation time (RT60), early decay time (EDT), and clarity metrics (C50/C80). These programs often generate waterfall plots and energy-time curves to visualize room acoustics.
Acoustic analysis software also provides tools for calculating speech intelligibility metrics like STI (Speech Transmission Index) and noise criteria curves. These measurements help evaluate spaces for specific purposes, such as classrooms or concert halls.
Challenges in Acoustic Analysis
Acoustic analysis techniques face several significant obstacles affecting measurement accuracy and interpretation. Environmental factors and computational demands represent the primary hurdles researchers and engineers must overcome.
Noise Interference
Unwanted background noise can severely compromise acoustic analysis results. Environmental sounds, equipment vibrations, and electrical interference often contaminate the target acoustic signal.
In voice analysis applications, background conversations, air conditioning systems, and recording equipment can introduce artifacts that skew measurements. These interferences are particularly problematic when analyzing subtle voice characteristics in clinical settings.
Recording studios minimize these issues using specialized isolation techniques, such as sound-absorbing materials and anechoic chambers. However, this level of control is rarely possible in field recordings or everyday clinical environments.
Digital filtering can help remove some noise, but distinguishing between noise and valuable data remains challenging. This is especially true when the noise’s acoustic properties overlap with the signal of interest.
Signal Processing Complexity
Acquiring and assessing acoustic signals involves complex mathematical operations and specialized algorithms. Fast Fourier Transform (FFT) and other spectral analysis techniques require significant computational resources.
Engineers performing acoustic analysis in structural systems must balance:
- Computational efficiency
- Model accuracy
- Time constraints
- Software limitations
Finding meaningful correlations between acoustic measurements and perceptual qualities presents another challenge. For example, researchers struggle to identify reliable acoustic markers corresponding to specific voice qualities that human listeners can easily detect.
The selection of appropriate parameters for analysis is not standardized across different fields. This makes it difficult to compare results between studies and establish universal benchmarks for acoustic phenomena.
Ethical Considerations
Conducting acoustic analysis requires careful attention to ethical standards, particularly regarding how voice data is collected, stored, and used.
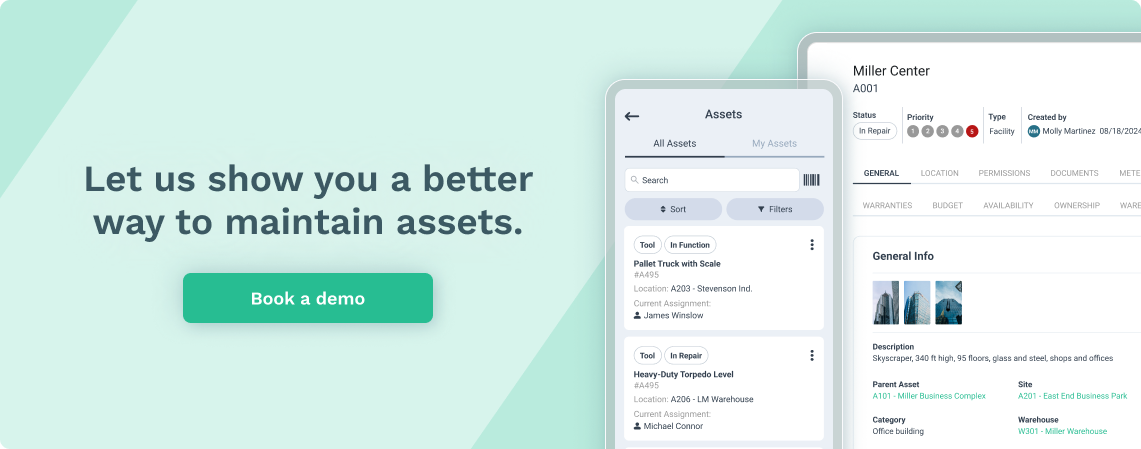
These considerations protect participants while ensuring research integrity.
Privacy in Sound Collection
Voice recordings contain personal and potentially identifiable information that demands proper protection. Researchers must obtain informed consent from participants and clearly explain how their voice samples will be used.
For clinical purposes, patients should understand whether their recordings will be used solely for diagnosis or research. This is especially important when analyzing pathological voices, as in studies comparing normal and abnormal voices.
Recording locations matter, too. Public versus private settings create different expectations of privacy. In public soundscape research, ethical listening practices require sensitivity to community needs and cultural contexts.
Researchers should minimize capturing identifying speech content when only acoustic properties are needed for analysis.
Data Security and Storage
Voice data must be protected through robust security measures. This includes encryption, password protection, and secure servers for all recordings.
Clear data retention policies are essential. Studies should specify how long recordings will be kept and whether they’ll be destroyed after analysis or archived for future research.
Anonymization techniques should be applied when storing voice samples. This might include:
- Removing identifying metadata
- Using code numbers instead of names
- Editing out personally identifying speech content
Access controls are critical. Only authorized personnel should be able to retrieve stored voice data, and tracking systems should document who accesses files and when.
Extra sensitivity is required when analyzing stress markers in voice as this data may reveal psychological states the participant didn’t intend to share.
Trends and Future Directions
Acoustic analysis technology is evolving rapidly, driven by innovations in machine learning and signal processing techniques. These advancements are changing how we collect, analyze, and interpret sound data across multiple fields.
Machine Learning in Acoustic Analysis
Machine learning has transformed acoustic analysis by enabling more accurate pattern recognition in sound signals. Traditional acoustic analysis relied heavily on manual interpretation, but instrumental acoustic measurements now use AI to detect subtle variations that humans might miss.
Deep learning algorithms can now identify pathological conditions from speech patterns in voice analysis with increasing accuracy. This technology helps medical professionals diagnose vocal nodules and polyps earlier through objective, reliable results.
Industries are adopting automated acoustic monitoring systems that use neural networks to detect equipment failures before they occur. These systems continuously analyze sound signatures and alert maintenance teams when abnormal patterns emerge.
The future points toward more personalized acoustic analysis tools that adapt to individual voices or equipment profiles, improving specificity and reducing false positives.
Advancements in Signal Processing
Modern signal processing techniques have significantly improved the quality and reliability of acoustic analysis. High-resolution spectral analysis allows a more detailed examination of sound components across various frequencies.
Researchers are developing new metrics using nonlinear dynamics to better understand complex acoustic phenomena. These methods capture chaotic aspects of sound that linear models miss.
Key innovations include:
- Wavelet transforms for time-frequency analysis
- Adaptive filtering techniques that reduce background noise
- Real-time processing capabilities for immediate feedback
Mobile technology has made acoustic analysis more accessible. Smartphones can now perform complex signal-processing tasks that once required specialized equipment.
Integrating these advanced signal processing methods with IoT devices creates new noise and vibration analysis possibilities in everyday environments.
Get a Free WorkTrek Demo
Let's show you how WorkTrek can help you optimize your maintenance operation.
Try for free