Get a Free WorkTrek Demo
Let's show you how WorkTrek can help you optimize your maintenance operation.
Try for freePredictive maintenance has become a game-changer for businesses looking to reduce downtime and maintenance costs.
Unlike traditional maintenance methods that fix problems after they occur, predictive maintenance uses data and technology to detect issues before equipment fails.
Building an effective predictive maintenance program involves analyzing critical assets, installing IoT sensors, and establishing baseline performance metrics to predict needed maintenance.
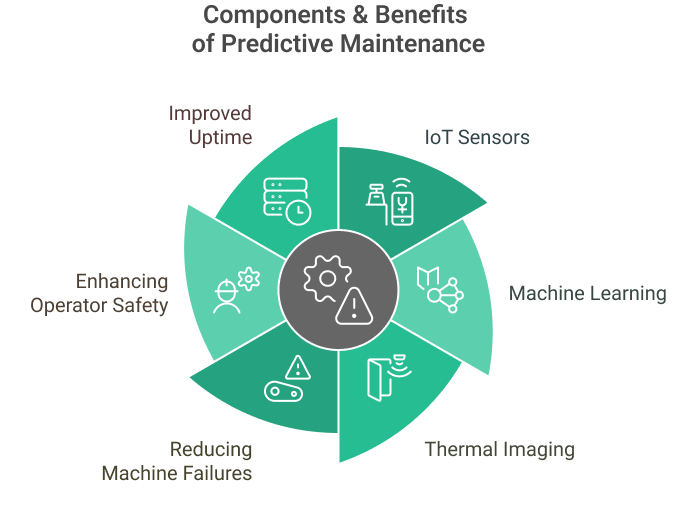
Getting started with a predictive maintenance program doesn’t have to be complicated. The process begins by identifying critical assets for PdM and understanding their failure patterns.
Companies then need to collect actionable data through sensors and monitoring systems. Many organizations find success by developing a pilot program to test their approach before scaling across their entire operation.
Key Takeaways
- Successful predictive maintenance programs require identifying critical assets, installing appropriate sensors, and establishing baseline performance metrics.
- Organizations should start with a small predictive maintenance pilot program before expanding their operations.
- Data collection and analysis form the foundation of effective predictive maintenance strategies that reduce downtime and extend equipment life.
Understanding Predictive Maintenance
Predictive maintenance is the opposite of reactive maintenance. A predictive maintenance solution leverages data, technology, and analytics to optimize maintenance activities and prevent unexpected failures.
Definition and Principles
Predictive maintenance is a strategy that monitors equipment conditions to determine when maintenance should occur. Unlike traditional approaches, it relies on real-time data from machines to predict failures before they happen.
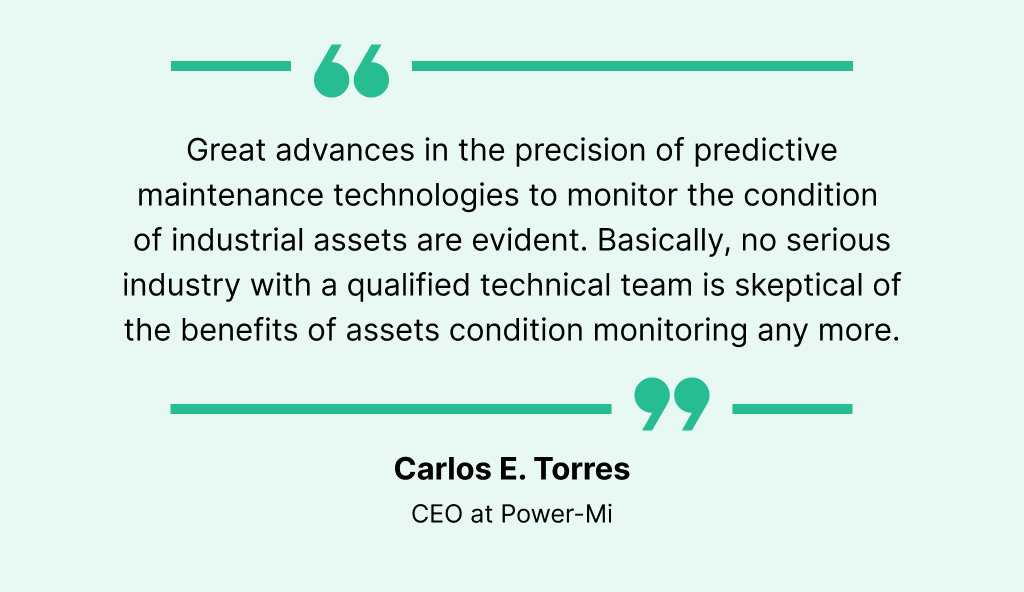
The core principles include:
- Condition monitoring: Using sensors to track parameters like vibration, temperature, and acoustics
- Data analysis: Applying algorithms to detect patterns indicating potential failures
- Just-in-time maintenance: Performing repairs only when needed, not on a fixed schedule
One predictive maintenance technique is installing IoT sensors and establishing baseline performance measurements.
These tools help technicians understand normal operating conditions, prioritize maintenance tasks, and recognize deviations that signal developing problems. They can also help perform maintenance and continuous online monitoring to improve equipment reliability.
The data-driven approach maximizes equipment availability and uptime while minimizing unnecessary maintenance interventions.
Benefits and Importance
Implementing predictive maintenance delivers significant advantages for organizations across industries:
Financial Benefits:
- Reduced maintenance costs (8% to 12% savings compared to reactive maintenance)
- Lower spare parts inventory requirements
- Extended equipment lifespan
- Decreased energy consumption
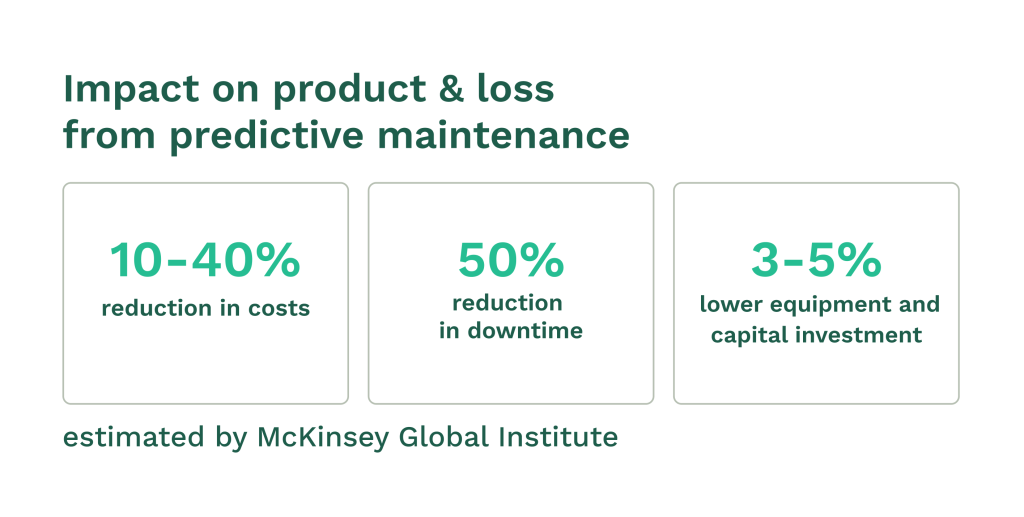
Operational Improvements:
- Minimized unplanned downtime (up to 50% reduction)
- 20% increase in labor productivity per a study by Deloitte
- Increased production reliability and quality
- Better allocation of maintenance resources
- Enhanced safety by preventing catastrophic failures
Predictive maintenance helps businesses move from crisis management to strategic planning. It transforms maintenance from a cost center into a value-adding function.
To measure predictive maintenance success successfully, focus on measuring overall equipment reliability and implement a data analysis strategy.
Predictive vs Preventative Maintenance
These two approaches represent different maintenance philosophies with distinct characteristics:
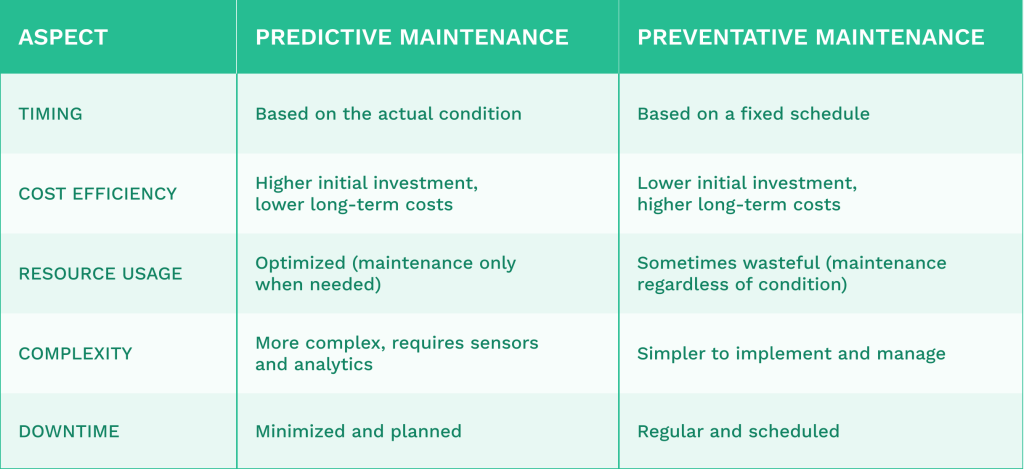
Preventative maintenance follows predetermined schedules regardless of equipment condition. This can result in unnecessary work on healthy components.
Predictive maintenance, however, uses data to determine the optimal time for maintenance activities. It accurately assesses actual equipment conditions to prevent premature interventions and unexpected failures.
Most successful organizations implement a hybrid approach, using preventative techniques for simple, low-cost items and predictive strategies for critical, expensive assets.
Key Components of a Predictive Maintenance Program
Implementing a successful predictive maintenance program relies on several components working together. These elements are combined to create a system that can effectively identify potential failures before they occur, saving time and resources—all designed to help improve asset reliability.
Data Collection and Management
To start, you need to ensure that you have a reliable data collection process in place. This forms the foundation of any predictive maintenance program.
Develop a process and system for gathering and storing information from multiple sources, including equipment sensors, maintenance records, and operational data.
Raw data from sensors can be noisy and has to be stored and cleaned in an accessible database format. Many companies use cloud-based systems that allow for real-time data access across departments.
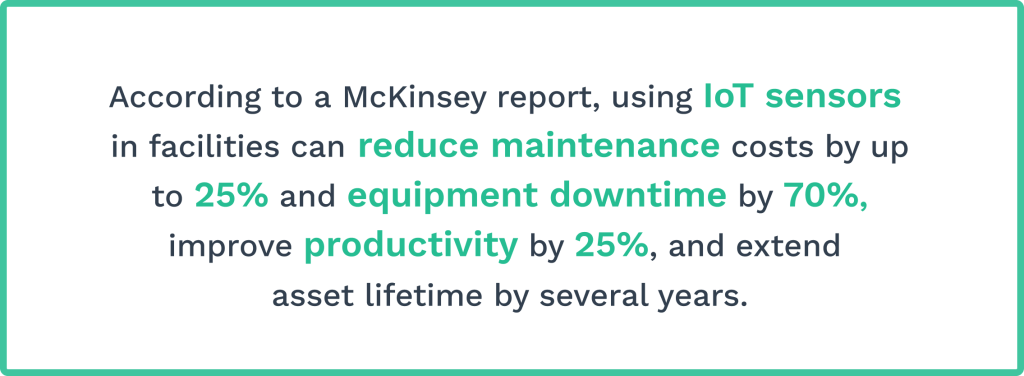
Data quality is critical—inaccurate readings can lead to false alarms or missed failures. Make sure to calibrate sensors regularly and validate their data integrity. When sensors are stored in production environments and exposed to excessive heat and debris, they can start reporting inaccurate data.
Sensor calibration and information validation help maintain data integrity.
Develop a baseline reading for normal operating conditions. This will provide a comparison point to identify deviations that might indicate developing problems.
Predictive Maintenance Technologies
Modern predictive maintenance employs various technologies to monitor equipment health. Vibration analysis can detect unusual movements that might indicate bearing wear or misalignment in rotating machinery.
Infrared thermography identifies hot spots in electrical systems or mechanical components that could signal impending failure.
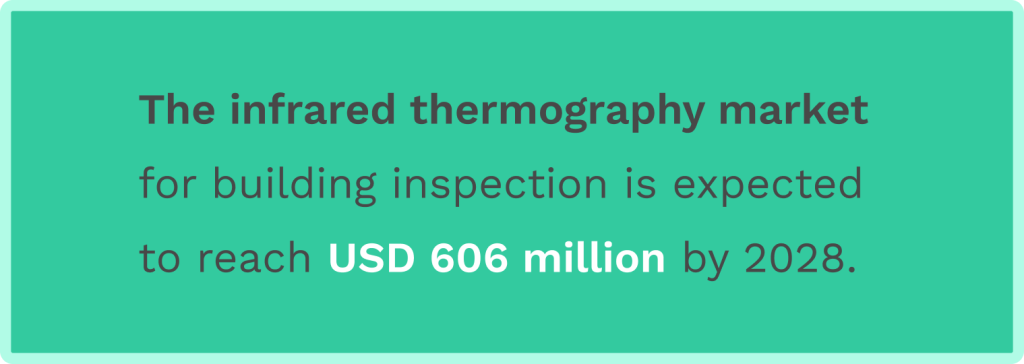
Oil analysis examines lubricant samples for contaminants that reveal internal wear patterns.
Ultrasonic testing can detect pressure leaks, electrical arcing, and bearing wear by capturing high-frequency sounds.
These technologies can vary in complexity and cost, so selecting the right tools is important to a successful predictive maintenance program.
Implementation should follow a phased approach, starting with critical assets and expanding as the program matures. Each technology requires proper staff training and certification to ensure correct usage and interpretation.
Condition Monitoring Equipment
Effective condition monitoring requires specialized equipment that is tailored to specific asset types.
It all starts with sensors that form the front line of monitoring—vibration sensors for rotating equipment, pressure transducers for hydraulic systems, and temperature probes for critical components.
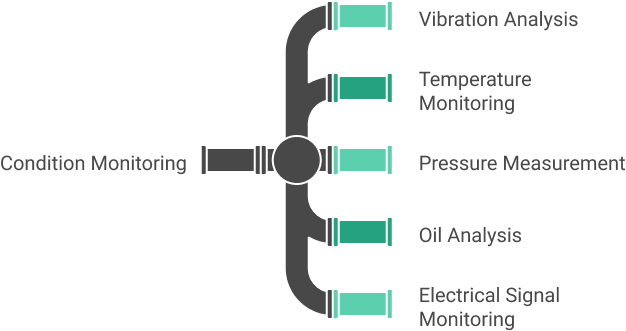
Portable data collectors enable technicians to gather readings during routine inspections. There are more advanced but costly systems that employ permanently installed sensors that continuously transmit data to monitoring systems.
The selection of monitoring equipment should be based on:
- Asset health & criticality
- Failure modes to be detected
- Environmental conditions
- Required monitoring frequency
- Budget constraints
Stay focused on the proper installation of these sensors. Incorrectly mounted sensors can provide misleading data or fail prematurely.
Don’t forget regular calibration to help maintain measurement accuracy and ensure reliable condition assessment.
Software and Data Analytics Tools
Analytics software transforms raw data into actionable intelligence—modern predictive maintenance platforms.
Data analysis software can integrate multiple data sources and apply advanced algorithms to identify patterns and anomalies.
Machine learning systems can detect subtle changes in equipment behavior that might escape human analysis. These systems improve as they process and train with more operational data and failure events.
Intuitive dashboards and KPIs provide at-a-glance status reports with color-coded alerts and trend visualizations.
Mobile applications allow maintenance teams to access critical information anywhere in the facility.
Integration with computerized maintenance management systems (CMMS) like WorkTrek or enterprise asset management (EAM) platforms streamlines work order generation when issues are detected.
This creates a closed-loop system where identified problems automatically trigger appropriate maintenance responses.
Developing a Predictive Maintenance Strategy
Focus on aligning your predictive maintenance strategy with technical capabilities and business objectives. It identifies critical assets, establishes metrics, and ensures proper resource allocation.
Asset Identification and Prioritization
The first step in developing a predictive maintenance strategy is identifying assets appropriate for predictive maintenance.
Using a predictive maintenance strategy for your equipment would waste resources.
Consider these criteria to prioritize assets:
- Criticality to operations: Equipment whose failure would halt production
- Replacement costs: Assets with high replacement or repair expenses
- Failure patterns: Equipment with predictable degradation patterns
- Safety implications: Machinery whose failure poses safety risks
After identification, historical failure data will be analyzed to understand patterns and frequencies.
This analysis should focus on determining which assets will provide the greatest return on investment and should be included in the PdM program.
Many organizations succeed by starting with a small subset of critical assets before expanding. This approach allows for process refinement before full-scale implementation.
Performance Metrics and KPIs
Establishing clear metrics is essential for measuring program effectiveness. Well-defined KPIs allow organizations to track progress and demonstrate value.
Common predictive maintenance KPIs include:
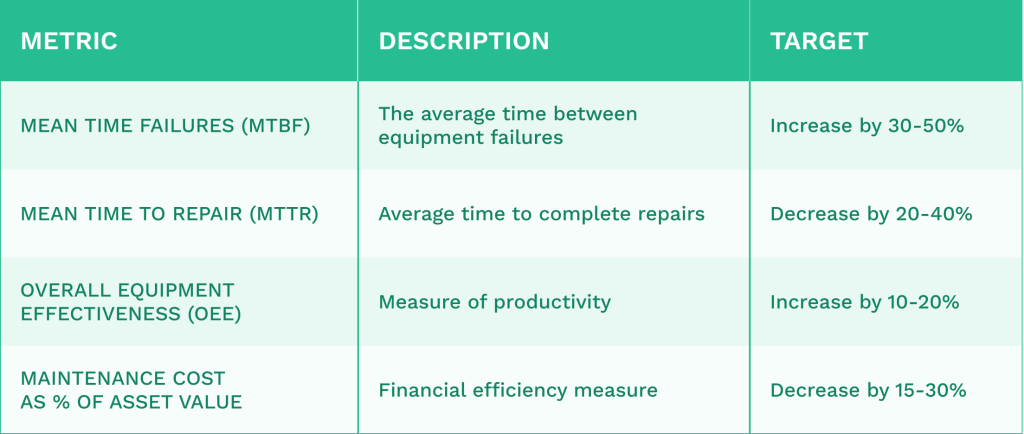
Organizations must establish baseline measurements before implementing PdM to track improvements accurately. This baseline data allows for meaningful comparisons.
Regularly reviewing these metrics helps refine the program and justify continued investment in predictive technologies.
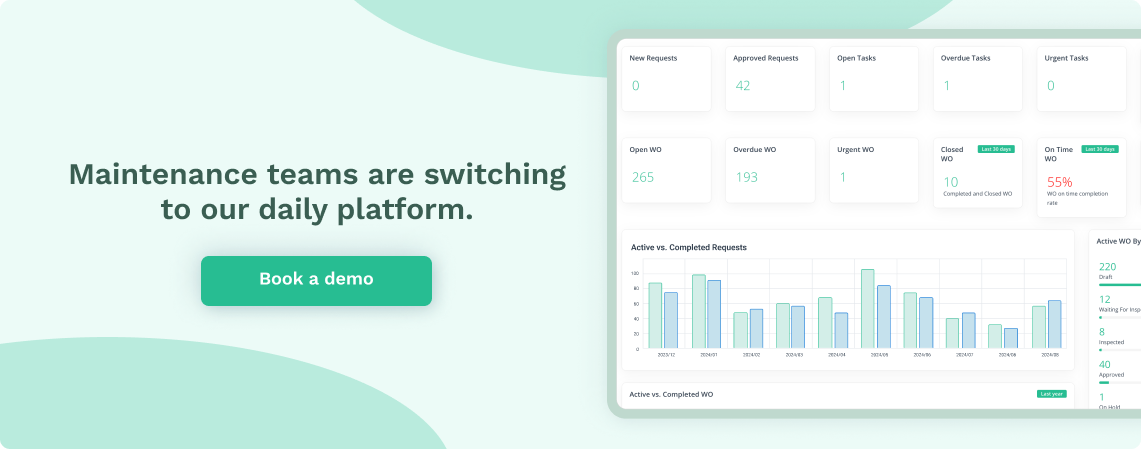
Resource Allocation and Training
Successful predictive maintenance requires appropriate resources and skilled personnel. Organizations must determine which functions to handle internally versus externally.
Key resource considerations include:
- Technology infrastructure: IoT sensors, data storage, analytics software
- Personnel: Data scientists, reliability engineers, maintenance technicians
- Budget: Initial investment and ongoing operational costs
- Time: Implementation timeline and maintenance schedules
Staff training is critical. Team members need to understand both the technology and methodology. This includes selecting proper condition monitoring equipment and interpreting the resulting data.

Cross-functional collaboration between maintenance, operations, and IT departments enhances program effectiveness. Clear responsibilities and communication channels should be established early in the development process.
Many organizations benefit from partnering with external specialists during initial implementation while building internal capabilities over time.
Implementation Process
You will need to create a plan before implementing a predictive maintenance program.
Below are a few suggested steps before fully implementing a PDM plan.
Planning and Scheduling
Start by identifying critical assets. Not all equipment needs the same level of monitoring – focus on machinery where failures cause significant downtime or safety risks.
Create a detailed timeline with specific milestones and responsibilities. This should include:
- Selection of appropriate monitoring technologies
- Installation timeframes
- Training schedules
- Review points
Establish clear metrics to measure success. These might include reduced downtime, maintenance cost savings, or extended equipment life. Your metrics should align with your organization’s specific goals.
Before you start the implementation process, consider your budget and ongoing costs. Consider budgets for both the initial implementation and ongoing support. Collecting actionable data requires investment in sensors, software, and training.
Integration with Existing Systems
Your predictive maintenance program should be complementary to current systems rather than replace them entirely. Assess compatibility with your existing predictive maintenance software, such as CMMS (Computerized Maintenance Management System).
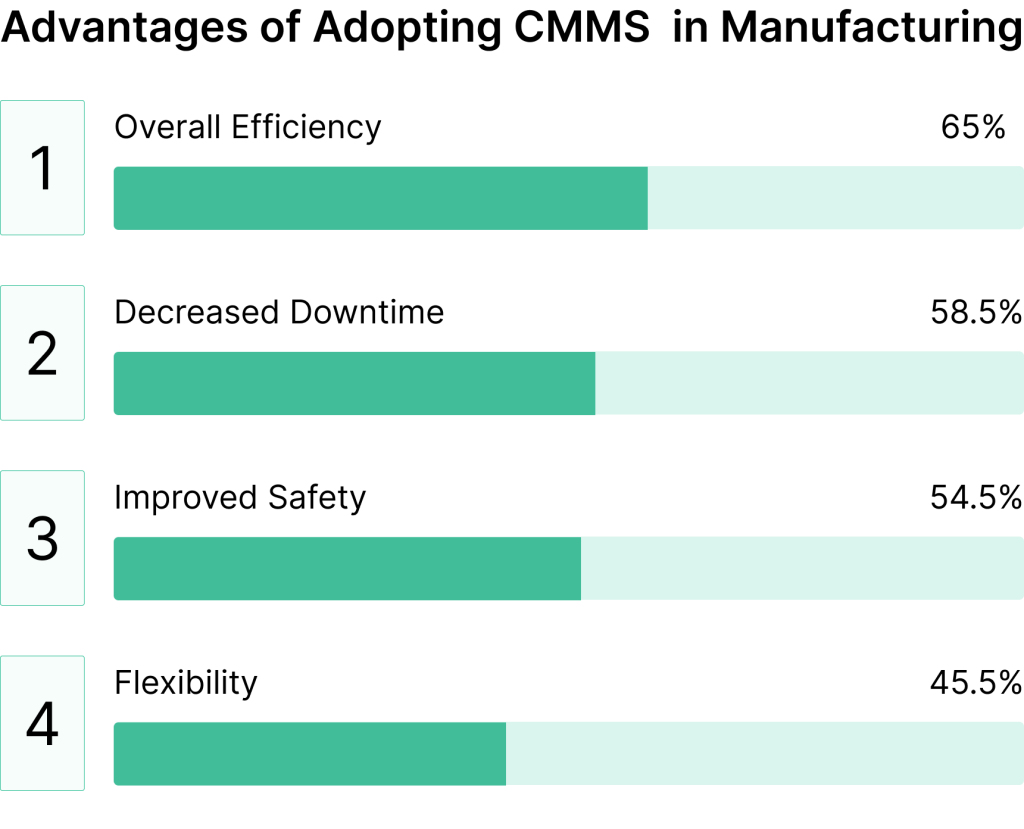
Data integration is critical for success. Create protocols for how predictive data flows into work order systems. This ensures that maintenance actions are triggered automatically when a potential failure is detected.
If direct integration isn’t possible, consider API connections between systems or middleware solutions. Many modern CMMS platforms offer open APIs and specific PdM modules that simplify this process.
Staff roles may need to be adjusted. Technicians will need training on both new technologies and changed workflows. Developing clear maintenance procedures helps smooth this transition.
Pilot Testing
Your predictive maintenance plan should include a small pilot program on selected assets before you scale predictive maintenance across the entire organization.
This allows you to refine your approach and demonstrate value quickly.
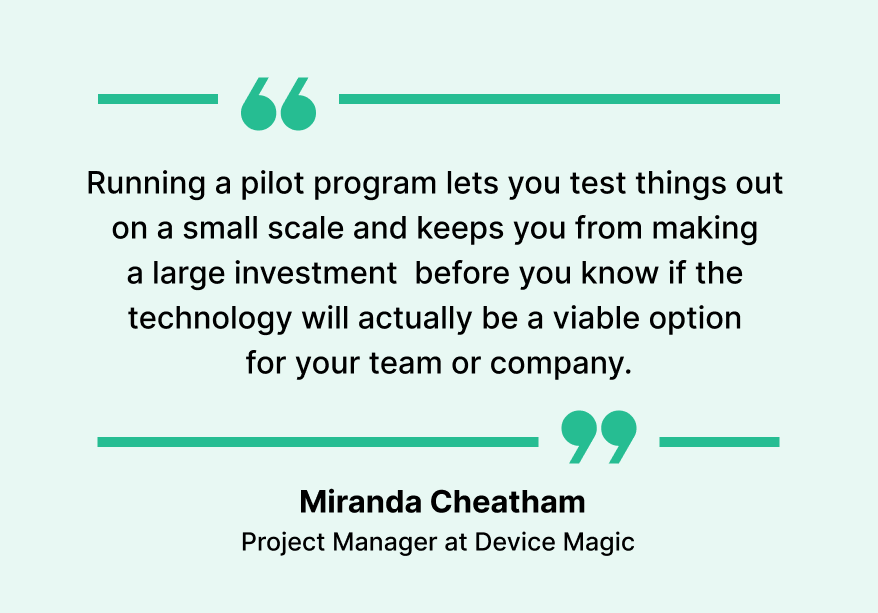
Select test equipment that:
- Has clear failure modes
- Is critical but not catastrophic if issues arise
- Represents typical assets in your facility
Set a specific timeframe for the pilot – depending on the size of your facility, this can take anywhere between 3-6 months. This provides enough data to evaluate effectiveness without delaying broader implementation.
Gather feedback from maintenance technicians and operators regularly during testing. Outline the benefits of predictive maintenance programs to their day-to-day operations.
Their insights will help identify practical issues that need to be addressed and any significant improvements that need to take place.
Document all successes and challenges encountered. This will create a blueprint for your full deployment and help justify the program to stakeholders.
Full-scale Deployment
Expand gradually based on lessons from your pilot testing. As you scale your predictive maintenance program, consider the lessons learned during the project’s pilot phase.
Develop a phased rollout plan that prioritizes:
- High-value assets
- Equipment groups with similar monitoring needs
- Locations with trained personnel
Remember continuous training during the project’s deployment phase. Create a support structure for the maintenance team, including a knowledge base, online training videos, and documentation.
Establish regular review meetings to evaluate program performance against your initial metrics. Be prepared to adjust your approach based on real-world results.
Document your implementation process thoroughly. This creates institutional knowledge that survives personnel changes and helps troubleshoot future issues.
Challenges and Considerations
Implementing predictive maintenance involves several hurdles organizations must overcome to realize its full benefits. Companies must address technical, financial, organizational, and regulatory aspects before transitioning from traditional maintenance approaches.
Data Quality and Quantity Challenges
Predictive maintenance programs face two primary data challenges: identifying key indicators for each asset and collecting sufficient quality data.
Many organizations struggle with inconsistent data collection methods and incomplete historical maintenance records.
Sensor data quality issues often emerge from:
- Poorly calibrated sensors
- Environmental interference
- Improper installation
- Data transmission failures
Legacy equipment may lack built-in sensors, requiring complex and expensive retrofitting.
Sensors tend to produce large amounts of data, so companies must address data storage and processing infrastructure challenges.
Data integration presents another hurdle, as maintenance information typically resides in various systems.
Creating a unified data model across multiple assets, manufacturers, and system types requires significant technical expertise and planning.
Cost-benefit Analysis
The transition to predictive maintenance requires substantial upfront investment in sensors, software, infrastructure, and training. Organizations must carefully weigh these costs against potential benefits.
Initial implementation costs typically include:
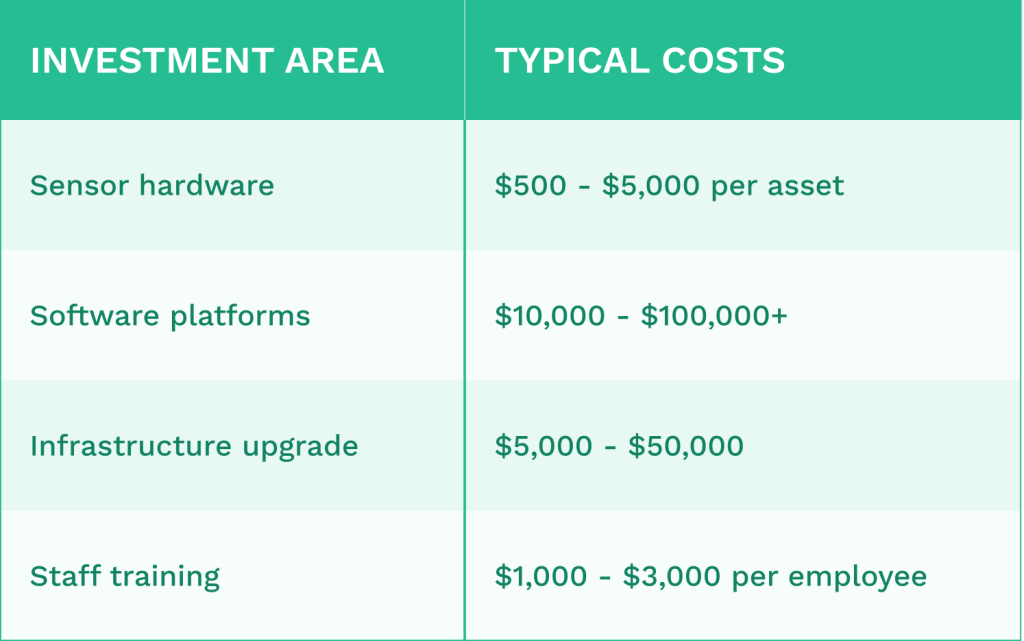
Return on investment timeframes vary significantly by industry, but most organizations see 12-24 months of payback periods. Companies should start with pilot programs on critical assets before full-scale implementation.
Maintenance teams must also consider ongoing costs, such as sensor maintenance, software subscriptions, and potential consulting fees for specialized analysis.
Change Management
Implementing predictive maintenance requires significant cultural and organizational changes. Resistance often occurs at multiple levels, from executives to maintenance technicians.
Maintenance staff may fear job displacement or lack confidence in new technologies. Providing comprehensive training and emphasizing how predictive tools enhance their expertise rather than replace it helps overcome resistance.
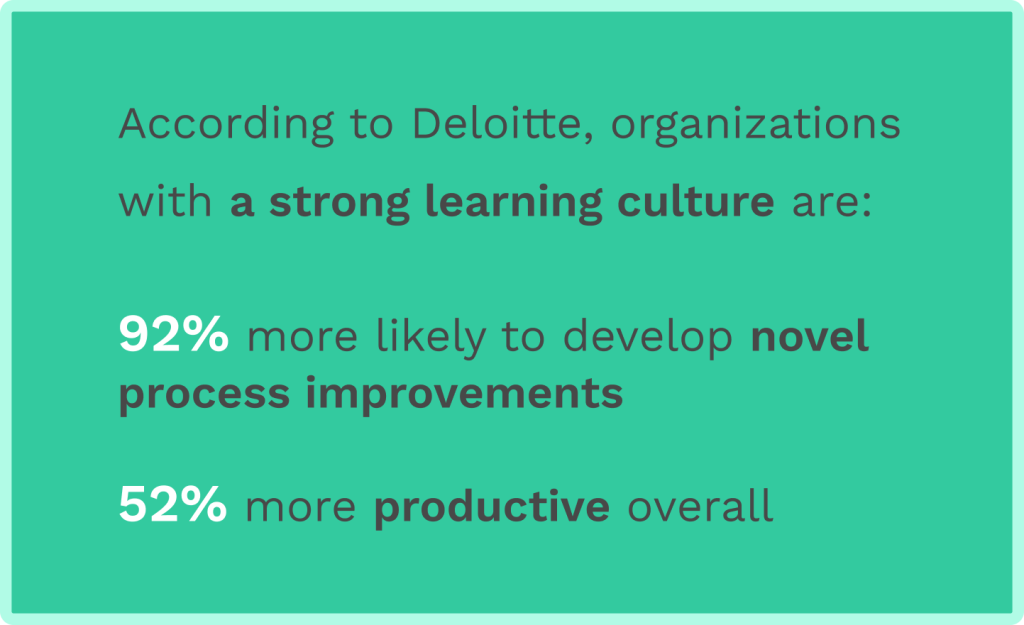
Management buy-in requires clear communication of benefits through:
- Specific ROI projections
- Case studies from similar organizations
- Pilot program results
Cross-departmental collaboration becomes essential as predictive maintenance spans IT, operations, and maintenance teams. Creating a dedicated task force with representatives from each area improves adoption rates.
Successful implementation requires establishing new workflows, responsibilities, and performance metrics that align with predictive approaches.
Regulatory Compliance and Safety
When implementing predictive maintenance systems, organizations must navigate various regulatory requirements.
Different industries have specific compliance standards governing data collection, storage, and equipment modifications.
When collecting large data sets, consider the privacy of this type of data. Work to establish clear data governance policies to address:
- Data ownership
- Access controls
- Retention periods
- Security protocols
Safety considerations arise when predictive systems interface with critical equipment or processes. Develop redundant safety mechanisms and validate that automation won’t create new hazards.
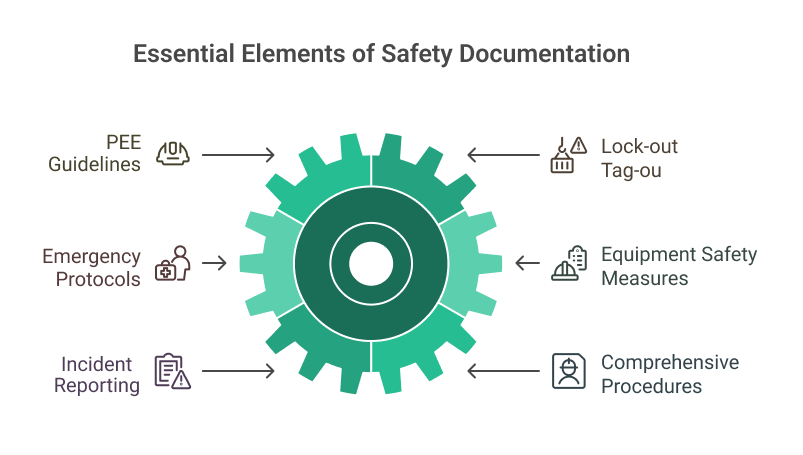
Documentation requirements increase with predictive systems. Teams must maintain detailed records of algorithms, model training data, and decision criteria to satisfy regulatory audits and potential liability concerns.
Maintenance Analytics and Improvement
The success of a predictive maintenance program depends on your ability to analyze data effectively and implement systematic improvements based on what you learn.
Data-driven insights enable maintenance teams to make better decisions and continuously refine their approach.
Predictive Analytics Techniques
Machine Learning Models form the backbone of modern predictive maintenance analytics. These models identify patterns in equipment data that indicate potential failures before they occur.
Common techniques include regression analysis, classification algorithms, and neural networks.
Time-series analysis helps track equipment performance over specific periods, making it easier to spot degradation patterns.
For example, gradual changes in vibration readings might indicate bearing wear that requires attention.
Key Analytical Methods:
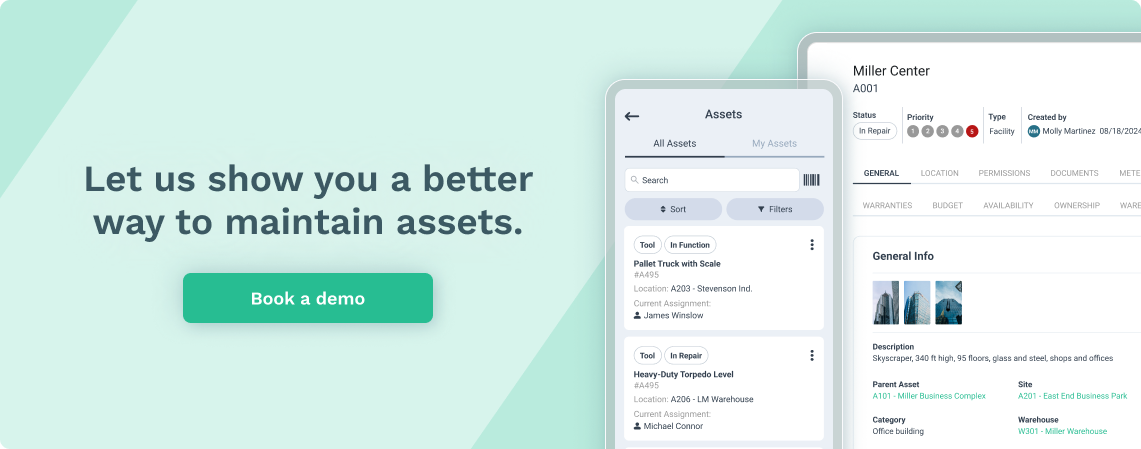
- Anomaly detection algorithms that flag unusual equipment behavior
- Failure mode prediction based on historical data
- Remaining useful life (RUL) calculations to optimize maintenance timing
- Condition indicators that track specific performance metrics
These techniques work best with domain expertise from maintenance professionals who understand equipment behavior.
Continuous Improvement Cycle
The PDCA (Plan-Do-Check-Act) cycle provides a structured framework for ongoing maintenance program enhancement. This approach ensures your predictive maintenance strategy evolves and improves over time.
Plan: Set clear objectives for your maintenance program and identify key performance indicators (KPIs) like mean time between failures or maintenance cost reduction targets.
Do: Implement your planned actions, collecting data throughout the process. This stage involves executing maintenance tasks based on predictive insights.
Check: Analyze results against your established KPIs to determine if your maintenance activities achieved the desired outcomes. This evaluation shows what’s working and what isn’t.
Act: Implement changes based on your analysis. This might involve adjusting sensor thresholds, updating prediction models, or refining your maintenance strategy.
Feedback Loops and Program Refinement
Effective feedback mechanisms ensure your predictive maintenance program captures valuable insights from every maintenance action. Regular review meetings help maintenance teams share observations that sensors might not capture.
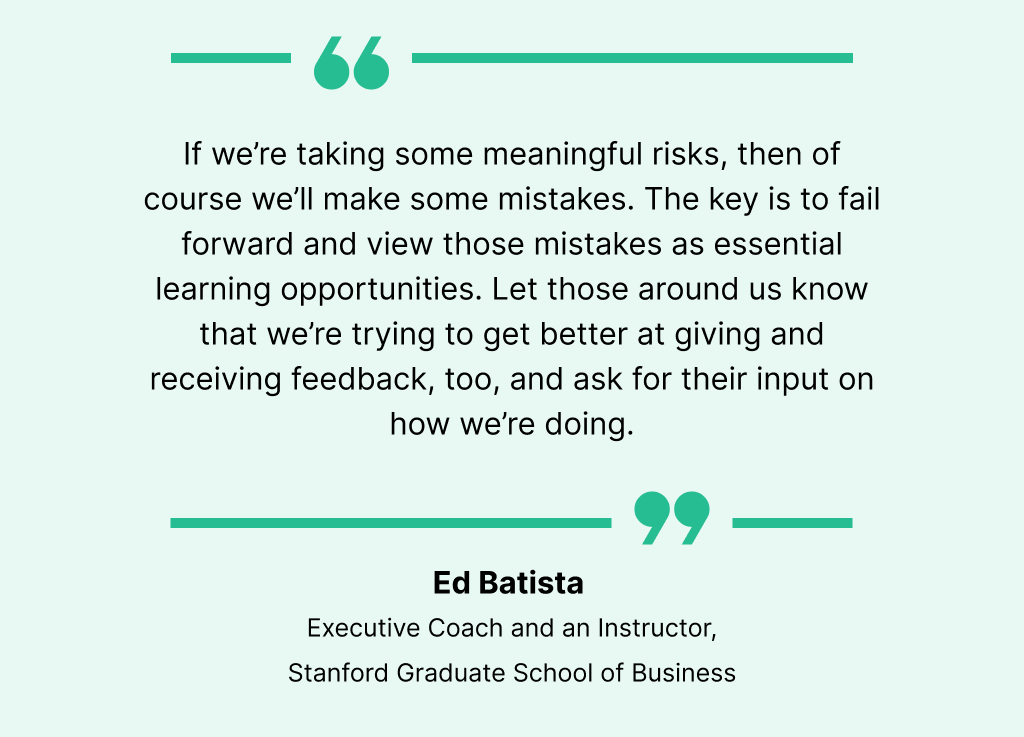
Documentation plays a crucial role in program refinement. Maintain detailed records of:
- Predicted failures vs. actual outcomes
- False positives/negatives in your prediction models
- Maintenance actions taken and their effectiveness
- Cost savings achieved through predictive interventions
Establishing regular review cycles helps identify areas for improvement. Monthly or quarterly assessments allow teams to evaluate prediction accuracy and adjust thresholds accordingly.
Cross-functional input improves program effectiveness. Operations, engineering, and maintenance personnel provide unique perspectives that enhance the overall maintenance strategy and ensure it aligns with broader business objectives.
Case Studies and Industry Applications
Examining real-world implementations of predictive maintenance shows how organizations across sectors achieve tangible benefits from these programs. These examples demonstrate practical applications, success metrics, and lessons learned that can inform your predictive maintenance strategy.
Manufacturing Sector
Manufacturing companies have embraced predictive maintenance to reduce costly downtime and extend equipment life. LSB Industries is a notable example of a company that has developed a successful predictive maintenance program that significantly improved equipment reliability.
In another case, a major automotive manufacturer implemented vibration analysis to detect bearing faults before failure. Their system identified unbalanced conditions and impact problems weeks before traditional methods would have caught them.
The ROI for these programs is compelling. Manufacturers typically report:
- 25-30% reduction in maintenance costs
- 70-75% decrease in breakdowns
- 35-45% reduction in downtime
Machine learning algorithms help production facilities monitor multiple variables simultaneously, creating early warning systems that allow maintenance teams to address issues during planned downtimes rather than critical production runs.
Energy and Utilities
Power generation and utility companies leverage predictive maintenance to ensure reliable service and manage remote assets efficiently. Wind farm operators use AI-powered systems to analyze turbine vibration patterns and weather data.
One electric utility implemented sensors across its grid infrastructure that monitor key performance indicators to predict potential failures. This approach:
- Reduced unplanned outages by 38%
- Extended equipment lifespan by 20%
- Optimized maintenance crew scheduling
Oil and gas companies deploy advanced analytics to monitor pipeline integrity. These systems correlate multiple data streams, including pressure, flow rates, and environmental factors, to predict potential leaks or failures before they occur.
The energy sector finds particular value in remote monitoring capabilities, which allow it to maintain widely distributed assets without constant physical inspections.
Transportation and Aviation
Airlines and transportation companies rely heavily on predictive maintenance to ensure safety and operational efficiency. Major airlines have implemented systems that analyze engine performance data in real time.
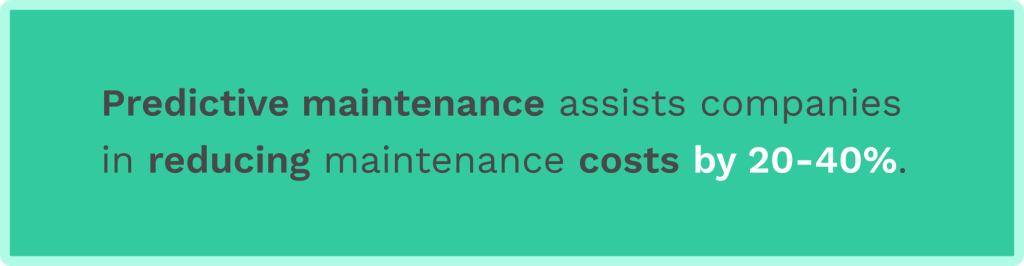
A commercial airline using machine learning algorithms to analyze fuel consumption patterns identified engine inefficiencies before they caused flight delays. Their program achieved:
- 16% reduction in maintenance-related delays
- $7-9 million annual savings in maintenance costs
- Improved fleet availability by 3.4%
Railway operators monitor track and railcar conditions through sensors that detect subtle changes in vibration and temperature patterns. This allows maintenance to be scheduled during low-traffic periods.
Fleet management companies use telematics data to predict vehicle component failures. By analyzing braking patterns, engine performance, and other metrics, they can schedule preventive maintenance before drivers experience problems.
Monitoring and Evaluation
Once your predictive maintenance program is up and running, develop an ongoing monitoring and evaluation. This monitoring type is essential for any PDM program’s long-term success.
Regular assessment helps identify areas for improvement and ensures the program delivers the expected return on investment.
Performance Tracking
Effective performance tracking requires establishing clear key performance indicators (KPIs) that align with your maintenance objectives. Common metrics include:
- Equipment uptime percentage
- Mean time between failures (MTBF)
- Reduction in unplanned downtime
- Maintenance cost savings
- Repair time reduction
Tracking these metrics can help you measure program effectiveness. Companies should collect pre-implementation baseline data and post-implementation results to measure improvements accurately.
Modern CMMS (Computerized Maintenance Management Systems) like WorkTrek can automate this tracking. Many organizations use dashboard visualizations to monitor KPIs in real time, allowing maintenance teams to identify performance trends quickly.
For critical assets, consider implementing more detailed tracking with specific thresholds that trigger notifications when performance metrics fall outside acceptable ranges.
Reporting and Analysis
Regular reporting transforms raw performance data into actionable insights. Data collection and analysis plans should be established early in the program development.
Reports should include:
- Current performance metrics compared to targets
- Trend analysis showing improvements over time
- Root cause analysis of any failures that occurred
- Cost-benefit analysis of the maintenance program
Visualization tools can really help make complex data more accessible. Charts showing failure rates before and after program implementation can demonstrate value to stakeholders.
Analysis should identify patterns that might indicate broader system issues. For example, if multiple assets show similar degradation patterns, this might point to operational or environmental factors affecting equipment longevity.
Cross-functional analysis meetings allow maintenance, operations, and management teams to review findings and develop coordinated responses.
Revisiting the Maintenance Strategy
A predictive maintenance program requires regular refinement to remain effective. Establishing a strategy is not a one-time event but an ongoing process.
Schedule quarterly reviews to evaluate:
- Effectiveness of current monitoring techniques
- Accuracy of failure predictions
- New technologies that could enhance the program
- Changes in production requirements or equipment usage
Be prepared to adjust sensor types and locations based on performance data. Sometimes, initial monitoring points prove less valuable than anticipated, requiring reconfiguration.
The maintenance team should also periodically reassess which assets are included in the predictive program. As equipment ages or production priorities shift, different assets may become more critical to operations.
Developing pilot programs to test new approaches allows for controlled evaluation before fully implementing changes to the maintenance strategy.
Conclusion
Implementing a predictive maintenance program can help optimize maintenance programs and improve equipment performance.
Utilizing predictive maintenance technologies, such as condition monitoring equipment and predictive analytics, can help businesses transition from reactive maintenance to a more proactive approach. This move helps reduce maintenance costs, minimize unplanned downtime, and extend critical assets’ life.
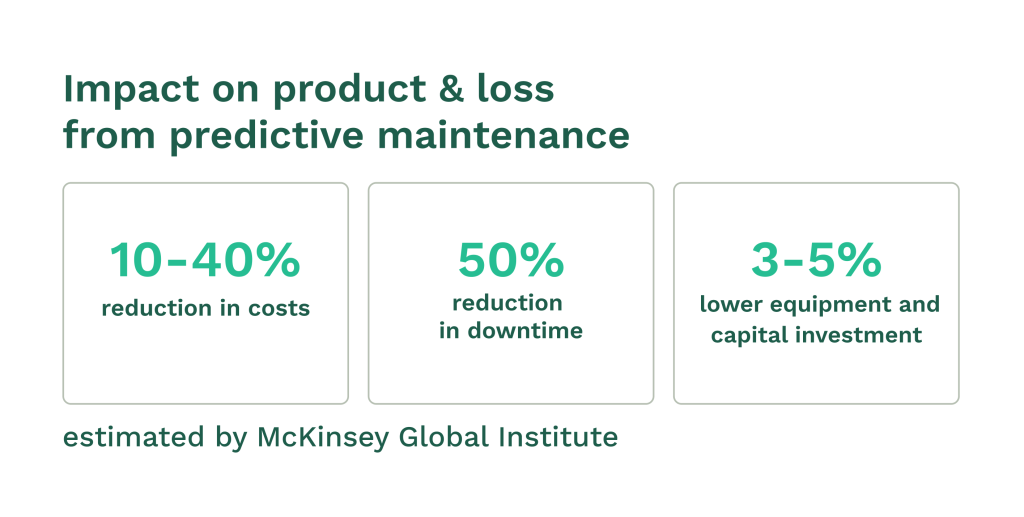
Starting with a predictive maintenance pilot program allows companies to test and refine their strategies, ensuring predictive maintenance success before scaling up.
Continuous monitoring and data analysis are crucial for identifying potential equipment failures and effectively prioritizing maintenance tasks.