Get a Free WorkTrek Demo
Let's show you how WorkTrek can help you optimize your maintenance operation.
Try for freeKeeping your equipment running smoothly is no longer about fixing things when they break. Smart companies now use predictive maintenance to spot problems before they happen.
The best predictive maintenance tools combine sensors, data analysis, and artificial intelligence to monitor equipment health, predict failures, and save businesses significant money on repairs and downtime.
Modern predictive maintenance has evolved far beyond simple scheduled check-ups. Today’s top company solutions offer sophisticated capabilities transforming maintenance from a cost center to a competitive advantage.
Additionally, successful companies have been increasingly deploying computerized maintenance management systems like WorkTrek to improve predictive maintenance outcomes.
Key Takeaways
- Predictive maintenance tools combine sensors, AI, and analytics to detect potential failures before they occur, reducing costly downtime.
- The market offers solutions for various needs, from enterprise platforms like GE Digital and Siemens to specialized tools for specific industries.
- Implementing the right predictive maintenance technology can transform maintenance from reactive to proactive, leading to substantial operational cost savings.
The Importance of Predictive Maintenance in Modern Industry
Predictive maintenance is a cornerstone technology modern industries use to optimize operations, improve operational efficiency, extend equipment life, and reduce costs.
Unlike traditional reactive maintenance, predictive maintenance uses data analysis to anticipate equipment failures before they occur.
One of the primary benefits is decreased downtime, which keeps production lines running smoothly. When machines unexpectedly fail, companies lose money every minute they’re not operational.
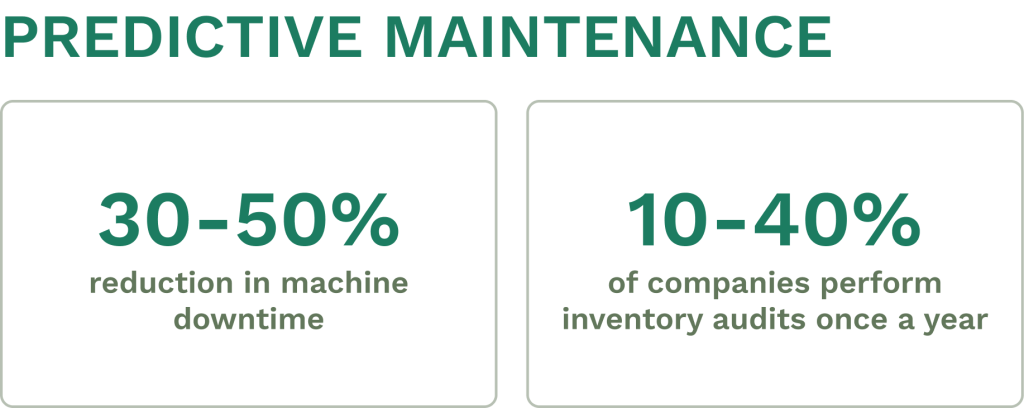
Lower maintenance costs represent another significant advantage. By addressing issues precisely when needed, businesses avoid the expenses of emergency repairs and unnecessary scheduled maintenance.
Predictive maintenance also contributes to increased equipment longevity. Machines that receive timely attention to developing problems tend to last longer than those subjected to reactive maintenance approaches.
Key benefits of predictive maintenance include:
- Reduced unexpected downtime
- Lower repair costs
- Extended equipment maintenance and lifespan
- Improved worker safety
- Enhanced product quality
- Better resource allocation
- Improved lifecycle management
- Improved equipment reliability
Modern predictive maintenance relies on condition monitoring tools and machine learning algorithms to detect potential failures. These technologies analyze patterns in equipment performance data to identify early warning signs.
Implementing predictive maintenance software helps teams effectively plan and execute maintenance tasks. These solutions coordinate activities and ensure that nothing falls through the cracks.
The manufacturing, energy, healthcare, and transportation industries increasingly adopt these sophisticated techniques to remain competitive in today’s fast-paced market environment.
Fundamentals of Predictive Maintenance Technology
Predictive maintenance relies on several key technologies that work together to anticipate equipment failures before they occur. These technologies gather data, analyze patterns, and identify potential issues systematically.
Condition Monitoring Techniques
Condition monitoring forms the backbone of predictive maintenance by continuously tracking equipment health. Vibration analysis is one of the most reliable techniques for detecting mechanical issues in rotating equipment.
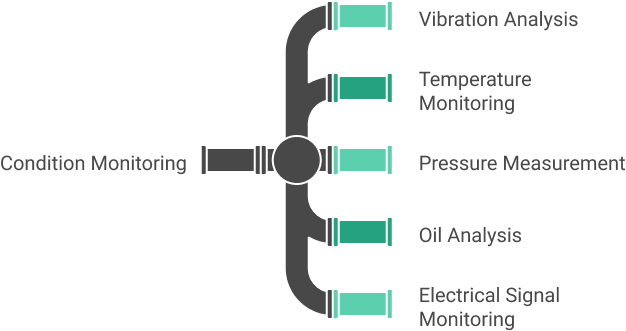
Other important monitoring methods include:
- Infrared thermography: Identifies overheating components by capturing heat signatures
- Ultrasonic testing: Detects air or fluid leaks and bearing failures by analyzing sound patterns
- Oil analysis: Examines lubricant samples for contamination and wear particles
- Motor current analysis: Measures electrical signals to identify motor degradation
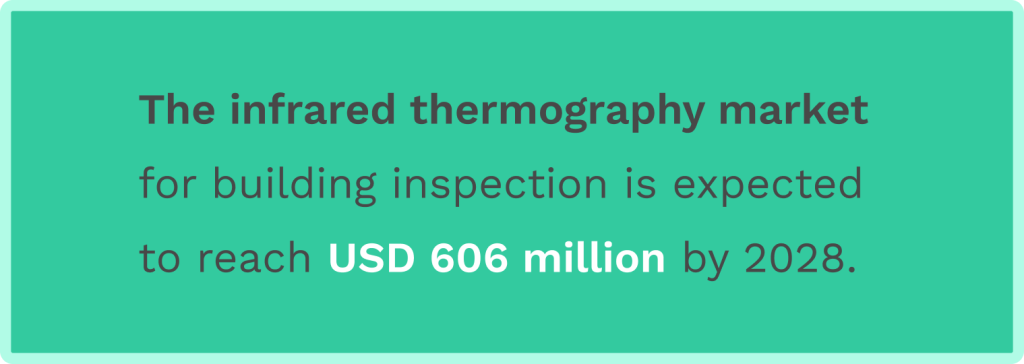
These techniques capture real-time data that serves as early indicators of potential failures. Modern sensors allow for continuous monitoring rather than periodic inspections, creating more comprehensive datasets.
Data Analytics and Machine Learning
The true power of predictive maintenance emerges when combining equipment data with advanced analytics.
Predictive maintenance software uses algorithms to identify patterns that precede failures.
Machine learning models analyze historical equipment data to:
- Establish normal operating parameters
- Detect anomalies that deviate from expected performance
- Predict remaining useful life of components
- Recommend optimal maintenance timing
These systems become more accurate over time as they process additional data. Leading solutions like GE Digital SmartSignal and Siemens Predictive Maintenance employ sophisticated algorithms that can detect subtle changes in equipment behavior weeks before failure.
Failure Mode and Effects Analysis (FMEA)
FMEA provides a structured framework for identifying potential failure points and their consequences. This systematic approach helps maintenance teams prioritize their efforts based on risk.
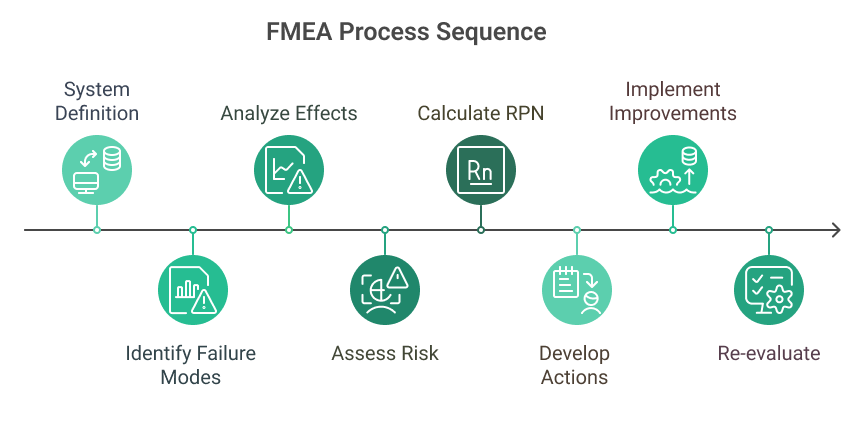
The FMEA process typically involves:
- Identifying all potential failure modes for each component
- Assessing the severity of each failure’s impact on operations
- Determining the likelihood of each failure occurring
- Calculating risk priority numbers (RPNs) to focus on highest-risk items
FMEA’s proactive detection of anomalies helps organizations avoid costly downtime. When integrated with predictive analytics, FMEA becomes even more powerful by connecting theoretical failure modes with equipment data.
This methodology requires cross-functional expertise from maintenance, operations, and engineering teams to succeed.
Don’t forget to regularly update your FMEA documentation to ensure it remains relevant as equipment and processes change.
Best Tools for Predictive Maintenance
The predictive maintenance landscape offers diverse tools that blend technology with practical applications. These tools help organizations detect problems early, reduce downtime, and optimize maintenance schedules through data-driven insights.
1. Predictive Maintenance Software Platforms
GE Digital SmartSignal and SAP Predictive Maintenance and Service lead the industry in providing comprehensive asset management solutions. These platforms use machine learning algorithms to analyze equipment data and predict failures before they occur.
PTC ThingWorx offers real-time monitoring capabilities that integrate with existing systems. The platform processes sensor data to identify patterns that indicate potential equipment issues.
Siemens Predictive Maintenance excels in manufacturing environments. It uses digital twins to simulate equipment performance, allowing maintenance teams to test scenarios before implementing changes on actual machinery.
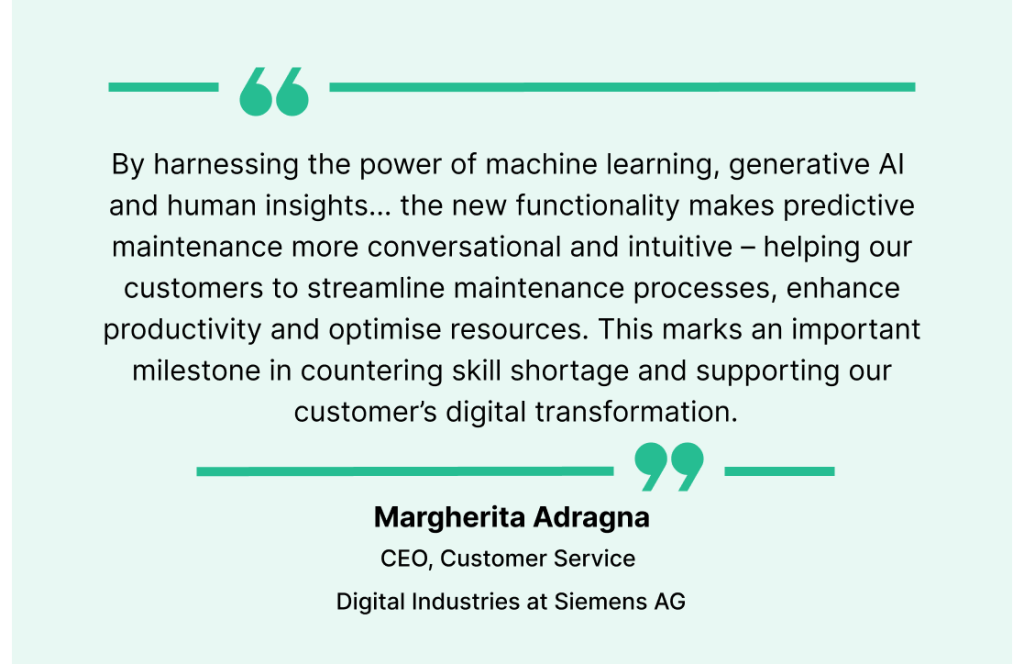
Other notable platforms include Uptake and Alteryx AI Platform, which focus on industrial applications with specialized analytics tools.
2. Wearable Technology and Sensors
Modern maintenance relies heavily on various sensor technologies that track critical equipment parameters. Vibration analysis sensors detect abnormal machine movements that often precede mechanical failures.
Temperature sensors monitor heat patterns in equipment, alerting teams to potential overheating issues. These are particularly useful in electrical systems and motors where heat often indicates problems.
Infrared analysis sensors identify hot spots in equipment that aren’t visible to the naked eye. Maintenance personnel wearing smart glasses can receive real-time alerts about equipment status during inspections.
Wearable devices also protect workers by monitoring environmental conditions and alerting them to dangerous situations. These sensors transmit data wirelessly to central monitoring systems for analysis.
3. Drones for Visual and Thermal Inspections
Drones with high-definition cameras provide access to hard-to-reach areas without risking worker safety.
They efficiently inspect tall structures, rooftops, and complex industrial equipment.
Thermal imaging drones detect heat variations in equipment, identifying potential issues before visible signs appear. This technology is particularly valuable for electrical infrastructure inspections.
AI-powered image recognition systems analyze drone footage to identify cracks, corrosion, or other defects. These systems compare current images with historical data to track degradation over time.
Drones reduce inspection costs by eliminating the need for scaffolding or lifts. They also increase inspection frequency, allowing more data collection and better predictive models.
4. Robotics for Automated Inspections
Autonomous robots navigate complex industrial environments to conduct routine inspections without human intervention. They excel in hazardous areas where human access is dangerous or limited.
Crawling robots inspect pipelines and confined spaces, using specialized sensors to detect leaks, corrosion, or structural weaknesses. Submersible robots perform similar functions for underwater infrastructure.
Wall-climbing robots inspect vertical surfaces in industrial settings using vacuum or magnetic attachments. They collect detailed visual and sensor data while maintaining steady contact with the surface.
Consistency in robotic inspections improves data quality for predictive models. Robots follow the same inspection patterns, eliminating human variability and creating reliable baseline measurements.
5. Acoustic Monitoring Devices
Ultrasonic analysis microphones detect high-frequency sounds emitted by failing equipment components. These sounds occur above human hearing range but provide valuable early warnings of issues.
Acoustic monitoring systems identify abnormal equipment sounds that indicate problems like bearing wear, steam leaks, or electrical arcing. The technology works well for rotating equipment like motors, pumps, and compressors.
Pattern recognition algorithms compare current sound signatures to normal operating conditions. Any deviation triggers alerts for maintenance teams to investigate potential problems.
Portable acoustic devices allow technicians to perform spot checks during regular maintenance rounds. Fixed systems continuously monitor critical equipment, creating long-term sound profiles for analysis.
6. Smart Maintenance Systems with IoT Integration
IoT sensors create connected networks of equipment that continuously transmit performance data. This enables real-time monitoring of entire facilities through centralized dashboards.
Cloud-based systems analyze this data stream using advanced algorithms to identify patterns and predict failures. These systems become more accurate over time as they learn from maintenance outcomes.
KONE stands out with its IoT-enabled elevator maintenance system that predicts issues before breakdowns occur. The system monitors thousands of data points per elevator to ensure reliability.
Smart maintenance systems automatically generate work orders when potential issues are detected. This streamlines the maintenance workflow and ensures timely interventions before failures occur.
7. Augmented Reality for Maintenance Training
AR headsets overlay digital information onto physical equipment, guiding technicians through complex maintenance procedures. This reduces errors and improves repair quality, especially for less experienced staff.
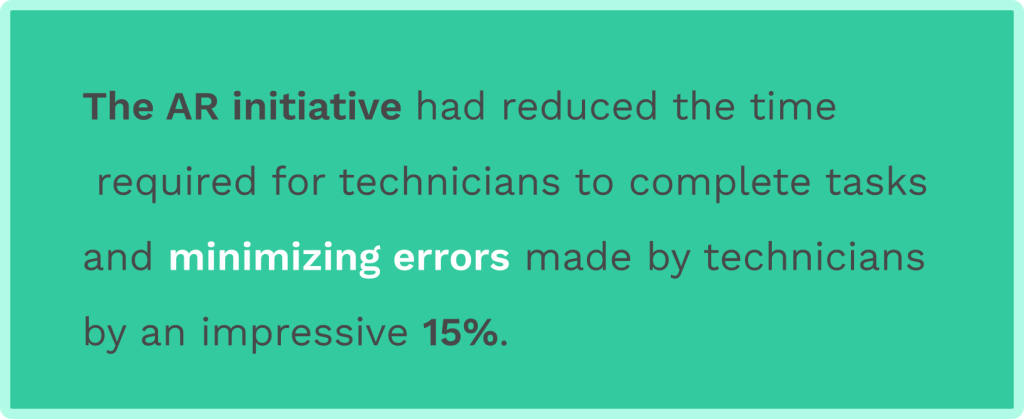
Remote expert assistance through AR allows specialists to see what technicians see in the field. They can provide real-time guidance by drawing annotations in the technician’s field of view.
Training simulations use AR to create realistic maintenance scenarios without risking actual equipment. Technicians practice procedures in a safe environment before working on critical systems.
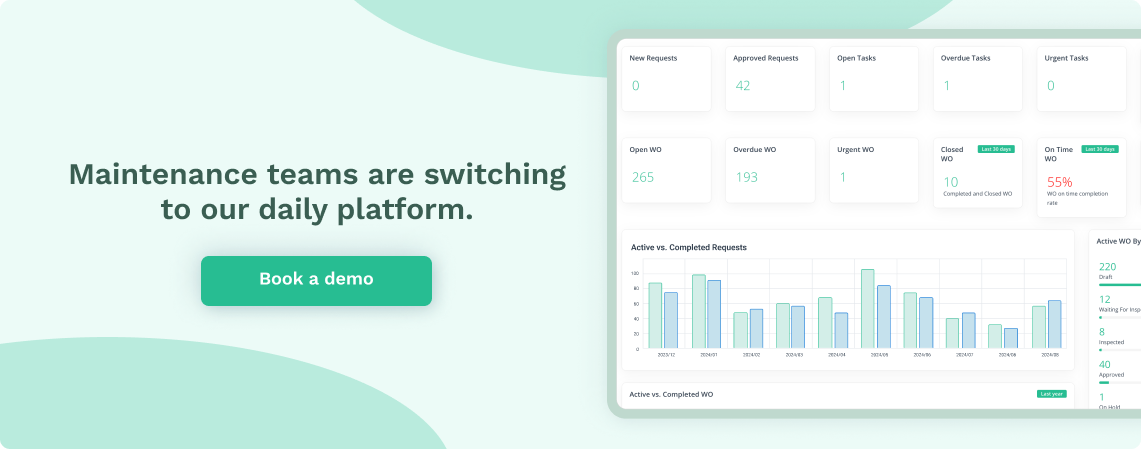
AR maintenance applications store equipment-specific information that technicians can access hands-free.
This includes repair histories, part specifications, and step-by-step maintenance procedures.
8. CMMS (Computerized Maintenance Management Systems)
A Computerized Maintenance Management System (CMMS) like WorkTrek transforms reactive maintenance into proactive strategies through several key capabilities.
CMMS platforms automate preventive maintenance scheduling based on time intervals or machine metrics, ensuring critical tasks are never missed. They provide customizable inspection checklists that standardize procedures and create accountability in the maintenance process.
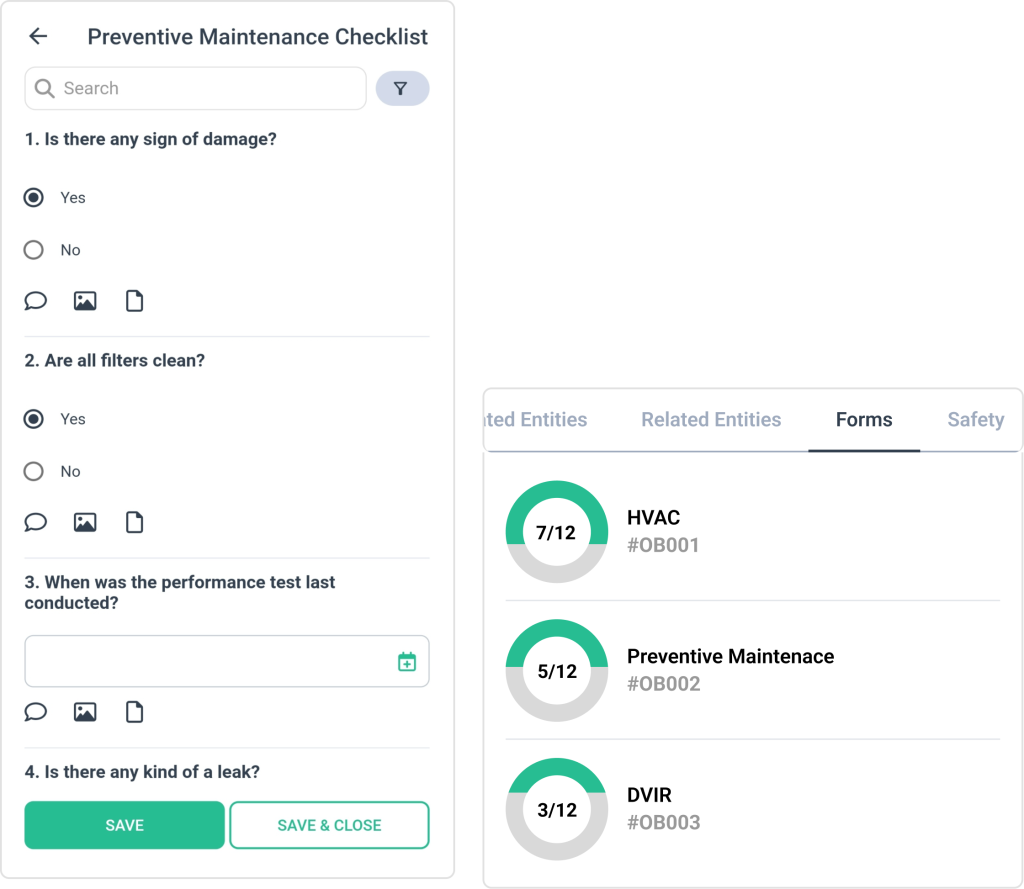
By maintaining comprehensive equipment histories, CMMS helps identify recurring issues before major failures occur. The work order management functionality streamlines maintenance activities through customizable templates for different maintenance types.
Inventory management features track spare parts and trigger reorder alerts when supplies run low. This ensures that necessary components are always available for scheduled maintenance work.
Performance analytics generate reports on metrics like schedule adherence and downtime reduction, helping teams continuously refine their proactive approach. Mobile applications allow technicians to access critical information in the field, supporting efficient documentation regardless of location.
Advanced CMMS solutions integrate with condition monitoring sensors, extending capabilities into predictive maintenance. This comprehensive approach shifts maintenance from reactive firefighting to strategic asset management, reducing downtime while extending equipment life.
Choosing the Right Predictive Maintenance Tools
Selecting an effective predictive maintenance solution requires careful evaluation of your specific operational needs, budget constraints, and technical infrastructure. The right tool must align with your industry requirements while delivering meaningful ROI and seamlessly integrating with existing systems.
Industry-Specific Considerations
Different industries face unique maintenance challenges that require specialized predictive maintenance solutions. Manufacturing facilities typically need tools for mechanical equipment, vibration analysis monitoring, and thermal imaging. Meanwhile, utilities benefit from solutions focused on grid reliability and outage prevention.
Healthcare organizations require predictive tools that meet strict regulatory compliance while maintaining critical life-support systems.
Transportation companies need tools to track vehicle fleet health and predict maintenance needs based on mileage and operating conditions. When evaluating vendors, look for industry-specific case studies to ensure they understand your sector’s unique challenges.
The most effective predictive maintenance implementations come from tools designed with your industry’s specific failure modes, compliance requirements, and operational constraints in mind.
Cost-Benefit Analysis
Implementing predictive maintenance tools requires initial investment, but the long-term benefits often outweigh these costs.
Consider direct expenses (software licenses, sensors, implementation) and indirect costs (training, system downtime during installation) when evaluating options.
The primary benefits include:
- Reduced unexpected downtime (typically 30-50%)
- Extended equipment lifespan (25-30% on average)
- Lower maintenance costs (25-30% reduction)
- Decreased spare parts inventory (20-25% reduction)
For most organizations, predictive maintenance tools can deliver ROI within 6-12 months. Calculate your potential savings by examining your maintenance expenses, equipment failure costs, and production losses from unplanned downtime.
Request vendor case studies with documented ROI figures from similar companies. This helps establish realistic expectations for your implementation and justifies the investment to stakeholders.
Ease of Integration
The most effective predictive maintenance solution must work seamlessly with your existing technology infrastructure.
Evaluate how each tool connects with your current maintenance management systems, ERP platforms, and control systems.
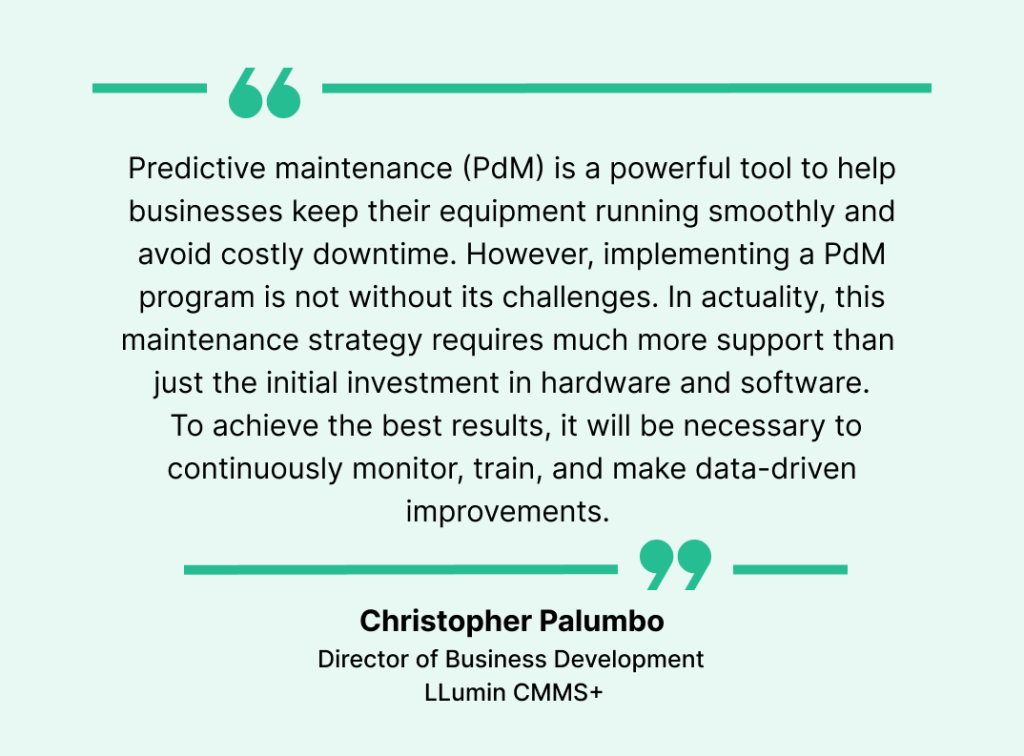
Key integration considerations include:
- API availability – Does the tool offer robust APIs for connecting your systems?
- Data compatibility – Can it process your existing sensor data formats?
- Implementation complexity – What resources are required for setup?
- Scalability – Will it grow with your operation’s needs?
Siemens Predictive Maintenance offers pre-built connectors for major industrial control systems, making integration substantially easier for manufacturing environments. Other solutions, such as SAP Predictive Maintenance, integrate naturally with existing SAP implementations.
Ask vendors about implementation timelines and required technical expertise. The best solution balances advanced capabilities with practical deployment requirements for your organization’s technical resources.
Vendor Support and Training
Comprehensive support and training are critical for successfully implementing and using predictive maintenance tools. Evaluate vendors based on their support structure, training programs, and customer success resources.
Look for providers offering:
- Dedicated implementation assistance
- 24/7 technical support options
- Comprehensive documentation
- Regular system updates and improvements
- On-site or virtual training programs
Maintenance software providers differ significantly in their support offerings.
Some licenses include basic support, while others charge premium fees for priority assistance. Consider these ongoing costs in your evaluation.
User training is particularly important for predictive maintenance tools. Staff need to understand not just how to use the software but also how to interpret the predictive data it generates. The best vendors provide initial training and ongoing educational resources as your team’s capabilities mature.
Implementing Predictive Maintenance in Your Operations
Turning predictive maintenance from concept to reality requires a structured approach that addresses strategy, skills, data, and ongoing improvement. Successful implementation depends on having the right foundations before deploying any tools.
Developing a Predictive Maintenance Strategy
Begin by identifying critical assets that would benefit most from predictive maintenance. These are typically equipment with high repair costs, significant safety impacts, or those that create bottlenecks when they fail.
Create objectives with measurable KPIs, such as reduced downtime, extended asset life, or lower maintenance costs. These metrics will help track your success.
Assessment and planning should include a comprehensive analysis of current maintenance processes to find gaps and improvement opportunities.
Develop a phased implementation roadmap rather than attempting to overhaul everything at once. Start with a pilot program on one critical system to demonstrate value before expanding.
Key considerations:
- Resource requirements (budget, personnel, time)
- Technology compatibility with existing systems
- Expected ROI timeframe
- Stakeholder buy-in from all levels
Training and Skills Development
Effective predictive maintenance requires both technical expertise and analytical capabilities. Team members need training in data collection, analysis, and interpretation of predictive models.
Create a comprehensive training program that addresses different skill levels and roles. Maintenance technicians need training that is different from that of data analysts or IT staff.
Consider bringing in external expertise initially. Consultants or vendors can provide specialized knowledge while internal team members develop their skills.
Required skill sets:
- Data analysis fundamentals
- Sensor technology understanding
- Specific equipment knowledge
- Software proficiency
- Root cause analysis techniques
Build a cross-functional team with maintenance, operations, IT, and management representatives. This diverse perspective helps ensure that all aspects of implementation are considered.
Data Collection and Management
Quality data is the foundation of any predictive maintenance program. Begin by identifying which data points truly predict equipment failure through data collection and acquisition.
Install appropriate sensors and monitoring equipment to capture real-time data on temperature, vibration, pressure, or other relevant parameters. Ensure these systems integrate with your existing infrastructure.
Establish data governance protocols that address:
- Data quality standards
- Storage requirements and duration
- Access controls and security
- Backup procedures
- Integration with other systems
Clean and normalize historical data before using it to train predictive models. Poor quality data leads to unreliable predictions and undermines confidence in the system.
Set up automated data collection to reduce manual entry errors and ensure consistent monitoring.
Continuous Improvement and Optimization
Predictive maintenance is not a set-it-and-forget-it solution. Regular performance reviews against established KPIs help identify what’s working and needs adjustment.
Schedule periodic evaluations of your prediction models’ accuracy. As equipment ages or operating conditions change, models may need recalibration or retraining.
Create feedback loops between maintenance teams and data analysts. Technicians can provide valuable insights about whether predictions match their real-world experience.
Real-time insights from your implementation can help minimize downtime and extend asset life beyond initial expectations.
Use these insights to refine your approach continuously.
Consider these improvement strategies:
- Add more data sources as you expand
- Refine prediction algorithms based on performance
- Adjust maintenance thresholds as you gain experience
- Incorporate new technologies as they become available
Document successes and failures to build institutional knowledge that survives personnel changes.
Challenges and Considerations for Predictive Maintenance
Implementing predictive maintenance involves several hurdles that organizations must navigate to achieve successful outcomes.
These range from data security concerns to technical limitations and the human factors involved in adoption.
Data Privacy and Security
Collecting vast amounts of equipment data raises significant privacy and security concerns. Organizations must protect sensitive operational data from unauthorized access and potential breaches.
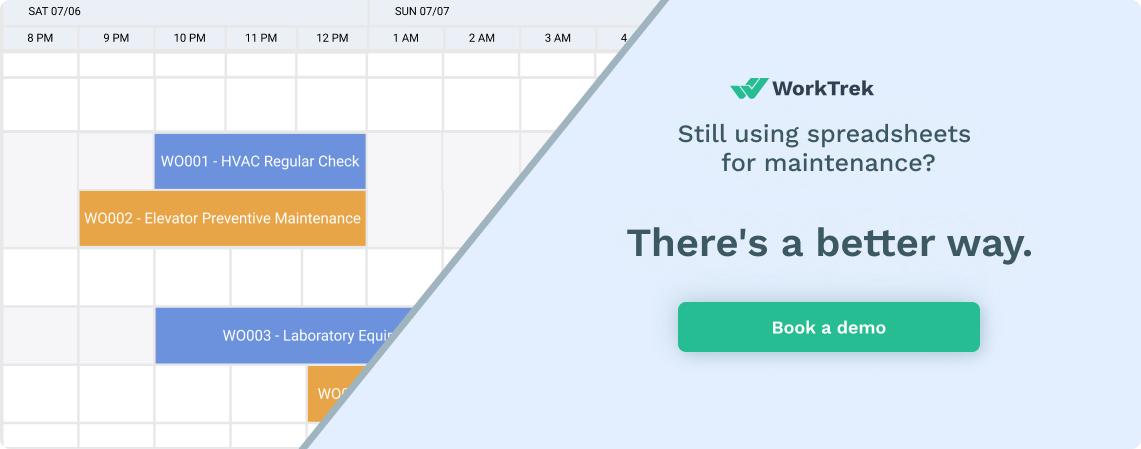
Predictive maintenance systems often require connectivity to external networks, creating potential entry points for cyber attacks. This increased attack surface necessitates robust security protocols and regular vulnerability assessments.
Many industries face strict regulatory requirements regarding data storage and processing. Companies must ensure their predictive maintenance implementations comply with regulations like GDPR, HIPAA, or industry-specific standards.
Key Security Measures:
- End-to-end encryption for data transmission
- Role-based access controls
- Regular security audits
- Secure API integrations
- On-premise solutions for highly sensitive environments
Technical Challenges and Limitations
One major hurdle is implementing system infrastructure, as organizations need the right tools and sensors to collect accurate data.
Older equipment often lacks built-in sensors, requiring retrofitting solutions.
Data quality issues can significantly impact analysis accuracy. Missing values, sensor drift, and measurement noise can lead to false positives or missed failure predictions.
Many organizations struggle with integrating predictive maintenance into existing systems. Legacy maintenance management software may not easily connect with newer predictive tools, creating data silos.
Another challenge is to identify key indicators for each asset. Different equipment types require unique monitoring parameters and failure thresholds.
Cultural Change and User Adoption
Transitioning from reactive or preventive maintenance to predictive approaches requires significant cultural shifts.
Maintenance teams accustomed to traditional methods may resist new technologies.
Training requirements can be substantial. Staff need to understand how to use new tools, interpret the data, and make informed decisions based on predictions.
Management buy-in is crucial for success. Predictive maintenance initiatives often fail to receive adequate resources or attention without leadership support.
Adoption Strategies:
- Involve maintenance teams early in implementation
- Demonstrate quick wins and ROI
- Provide continuous training opportunities
- Create clear escalation paths for alerts
- Recognize and reward adoption
Maintenance Scheduling and Planning
Balancing predictive alerts with existing maintenance schedules creates logistical challenges. Organizations must determine when to act on predictions versus following established maintenance intervals.
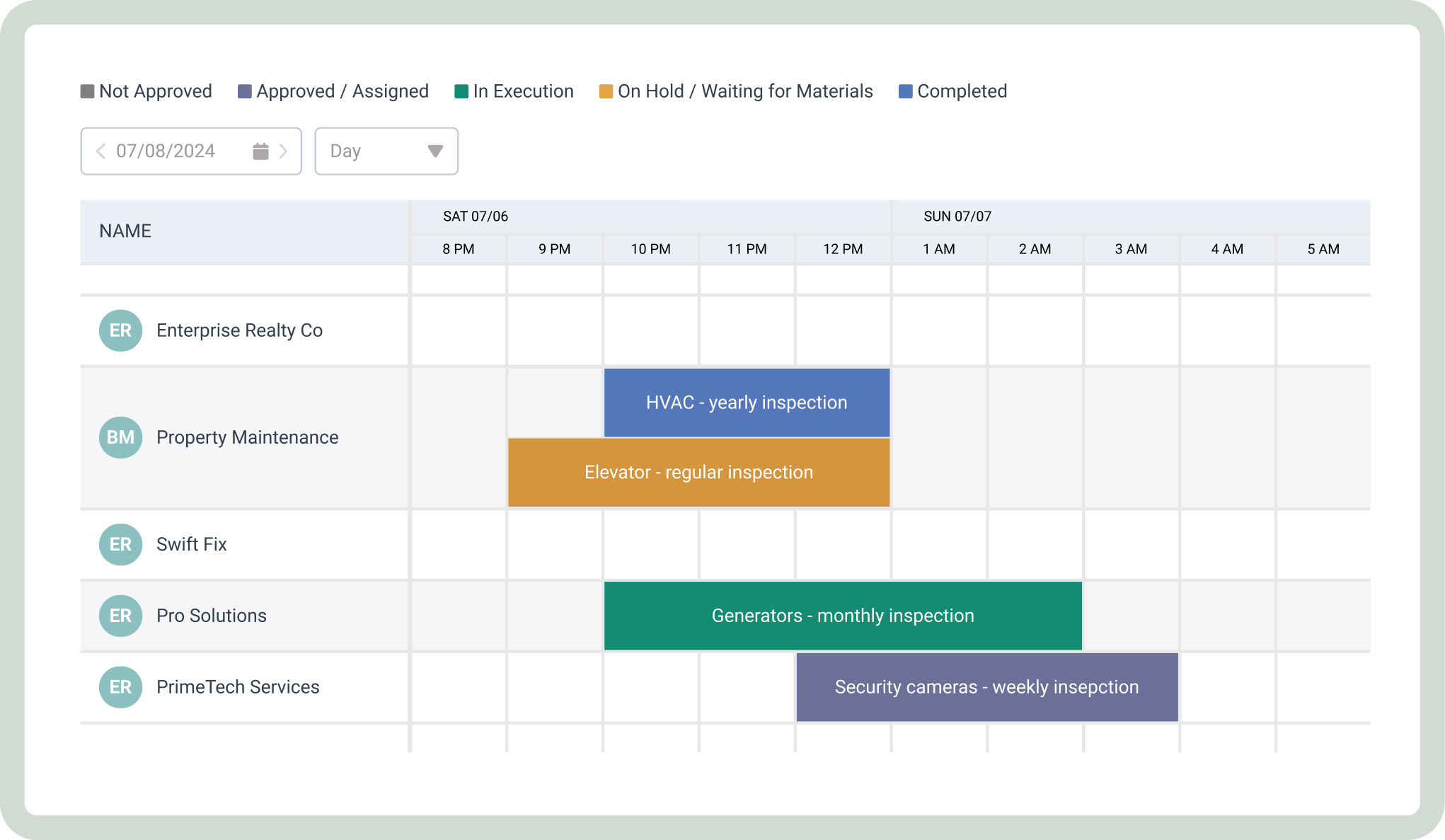
Predictive systems make resource allocation more complex. Maintenance teams need flexibility to respond to algorithmic recommendations while managing routine work.
Integration with maintenance management systems is essential for effective planning. Work orders generated from predictive alerts should flow seamlessly into existing workflows.
Equipment downtime coordination requires careful planning. Even with a warning, taking critical assets offline for maintenance impacts production schedules and requires cross-departmental coordination.
The Future of Predictive Maintenance Tools
Predictive maintenance is evolving rapidly, and technological innovations are reshaping industrial operations.
These advancements focus on smarter analytics, deeper integration with manufacturing systems, and contributions to environmental sustainability.
Advancements in AI and Machine Learning
The next generation of predictive maintenance tools will feature significantly more sophisticated AI capabilities. Machine learning algorithms are becoming increasingly accurate at detecting subtle equipment anomalies before they cause failures.
Deep learning models will soon analyze complex patterns across multiple data streams simultaneously, recognizing issues that current systems might miss. These advances will reduce the false positives that plague many current solutions.
Edge computing integration will enable direct real-time processing of industrial equipment. This eliminates latency issues and allows for immediate response to developing problems.
Natural language processing will make these tools more accessible to maintenance staff without technical expertise.
Workers can query systems using plain language and receive actionable insights rather than complex data.
Integration with Smart Factory Concepts
Predictive maintenance is becoming a cornerstone of Industry 4.0 implementations. Future tools will seamlessly connect with entire smart factory ecosystems rather than functioning as standalone solutions.
Digital twin technology will create virtual replicas of physical assets, allowing for simulation-based maintenance planning. These simulations will test different maintenance scenarios before implementing them in the real world.
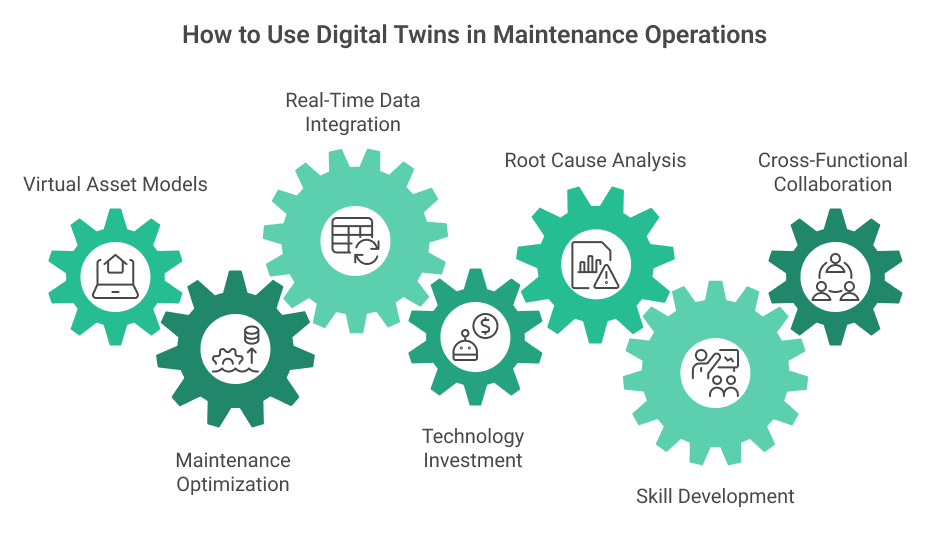
Interoperability standards are evolving to ensure different platforms can share data easily. This will eliminate the information silos that currently limit comprehensive equipment monitoring.
Augmented reality interfaces will guide technicians through maintenance procedures with visual overlays on actual equipment.
This will reduce training requirements and improve repair quality.
Predictive Maintenance and Sustainability
The predictive maintenance market is increasingly focusing on sustainability benefits. Future tools will quantify energy savings and reduced material waste from optimized maintenance schedules.
Equipment lifespan extensions will be measured and reported as sustainability metrics. Organizations will use these metrics for environmental compliance reporting and sustainability initiatives.
Carbon footprint calculations will be integrated into maintenance planning. Algorithms will prioritize maintenance activities that provide the most significant environmental benefit alongside operational improvements.
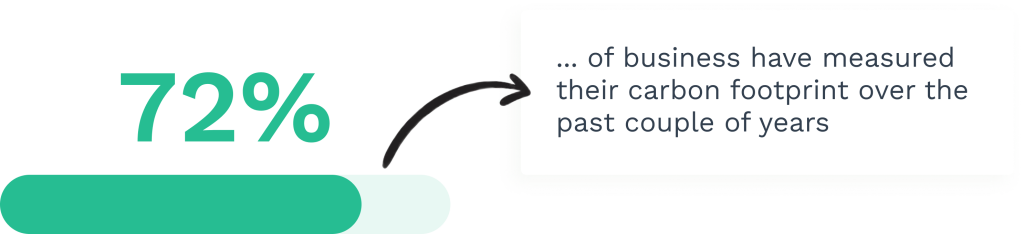
Resource optimization features will go beyond preventing failures to ensure maximum efficiency. Systems will recommend adjustments to operating parameters that reduce energy consumption while maintaining production targets.
Conclusion
In conclusion, integrating predictive maintenance tools and technologies revolutionizes how industries manage equipment health and reliability.
By leveraging advanced data analytics, machine learning, and condition monitoring, businesses can predict equipment failures before they occur, significantly reducing downtime and maintenance costs.
Adopting these tools extends equipment life and enhances operational efficiency and resource allocation, transforming maintenance from a reactive to a proactive strategy.
The future promises even more sophisticated AI capabilities, seamless integration with smart factory ecosystems, and a stronger focus on environmental sustainability. By embracing these advancements, organizations can stay competitive, improve asset performance, and contribute to a more sustainable future.